January 23, 2025 · 20 min read
Improving Investment Decision-Making with AI Meeting Analytics
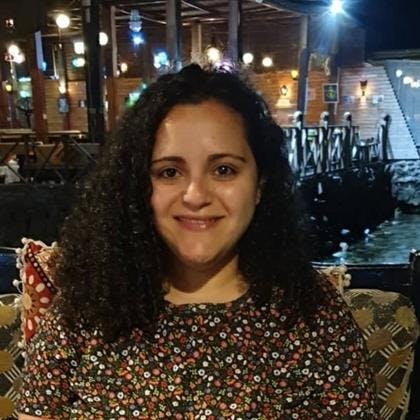
Shaimaa Badawi
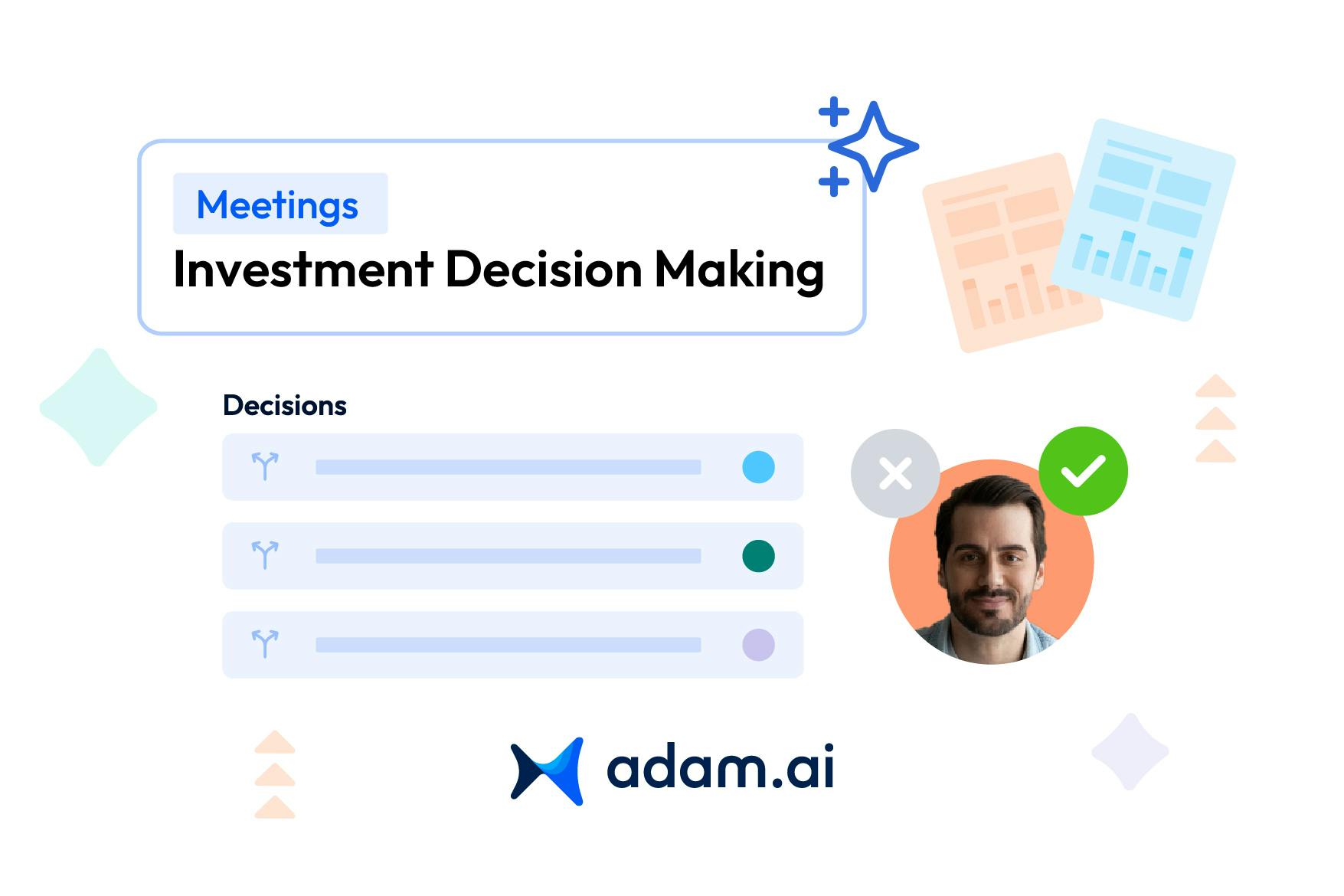
Investment decision-making are not just about market data and financial models. The way investment teams discuss, strategize, and follow through on key decisions plays a crucial role in portfolio success. Without structured meetings and clear action tracking, valuable insights can get lost, leading to missed opportunities and unmanaged risks.
This article explores how AI-powered meeting analytics help investment teams organize discussions, collaborate in real time, track decisions, and analyze meeting data to improve financial strategies.
What is investment decision-making, and why is it crucial for enterprises?
Investment decision-making involves selecting where to allocate funds, such as stocks, bonds, real estate, or new projects, to align with an organization’s long-term goals. It’s not just about chasing profits; it’s about balancing potential returns with the risks involved. For instance, choosing whether to invest in a new software product or to acquire an existing competitor can have vastly different financial and strategic outcomes.
Why it’s crucial for enterprises
1. Guarding against risks
Every investment carries uncertainty. You need to weigh potential rewards against market fluctuations, regulatory shifts, and economic downturns. Sound decision-making ensures you don’t overextend resources, helping your organization remain financially stable.
AI-driven tools are revolutionizing compliance risk management by automating regulatory monitoring, reducing human error, and ensuring financial decisions align with legal frameworks.
2. Driving sustainable growth
Sitting on capital rarely propels a business forward. Investing in technology, talent, or infrastructure can spark innovation and strengthen your competitive position. Organizations that consistently back the right projects often adapt better to changing conditions and keep ahead of industry trends.
3. Protecting financial stability
Smart investments help maintain liquidity and keep debt under control. On the other hand, a poorly timed or misaligned investment might drain cash flow or force you to cut back on essential operations.
By focusing on careful capital allocation, you safeguard your company’s day-to-day and long-term viability. AI enhances financial reporting for boards by providing real-time insights, automating report generation, and ensuring transparency in investment decisions.
4. Aligning with strategic goals
Not every move is about quick gains. Some enterprises direct funds toward sustainability or research and development to ensure they stay relevant in the future. These investments might not pay off immediately, but they can fortify your market position over time.
5. Navigating volatile markets
Economic and political factors often shift with little warning. When you rely on data-driven analyses, like market trends and competitor moves, you’re better equipped to pivot quickly, hedge against downturns, and seize emerging opportunities.
Organizations that leverage AI for financial decision-making also gain an edge in enhancing customer experience in financial services by offering personalized recommendations and faster response times.
Approaches to investment decisions
- Capital budgeting: Weighing the feasibility of large-scale investments, such as acquiring a smaller firm or launching a major product line.
- Portfolio management: Balancing various assets (e.g., stocks, bonds, real estate) to achieve steady returns while limiting exposure to a single market risk.
- Risk assessment models: Examining current and projected economic indicators, as well as competitor actions, to make more informed choices.
How does AI enhance investment decision-making compared to traditional methods?
Investment decision-making once relied on financial data, market analysis, and economic forecasting, often handled by manual processes and human expertise. While these methods offered valuable insights, they came with limitations such as slow data processing, human bias, and difficulty analyzing large volumes of unstructured information. This is where AI offers a new advantage.
1. Faster, more accurate data processing
Traditional investment analysis can involve reviewing countless financial statements and market indicators by hand. AI processes millions of data points in seconds and pulls from sources like earnings reports, stock market data, and even social media sentiment.
Example: AI-powered trading platforms detect micro-trends almost instantly, allowing you to react to market shifts faster than a human analyst ever could.
2. Smarter risk assessment and predictive analytics
Human analysts often rely on historical data and visible risk factors. AI digs deeper, finding hidden patterns and predicting potential downturns before they become obvious.
Example: AI-driven predictive models gauge the likelihood of a company defaulting or its stock price dropping by examining industry trends, global economic shifts, and even tone-of-voice analysis from earnings calls.
3. Reducing human bias in decision-making
Emotional attachment to certain assets or overconfidence in past strategies can cloud judgment. AI bases decisions purely on data, helping you make more objective calls.
Example: A manager might hold onto an underperforming stock out of loyalty, while an AI-powered system would sell it as soon as real-time data indicates it’s no longer viable.
4. AI-powered sentiment analysis for market insights
Traditional methods focus on fundamental and technical analysis. AI goes further by scanning news articles, social media posts, and analyst reports to gauge public perception.
Example: AI can detect growing negativity around a stock, such as skepticism in a CEO’s earnings call, well before share prices react, giving you time to adjust your position.
5. AI-driven portfolio optimization
Managing a portfolio requires continuous oversight and risk balancing. AI adapts to changing market conditions in real time, rather than following static, predefined rules.
Example: Robo-advisors powered by AI analyze economic indicators and your risk profile, automatically rebalancing assets to capitalize on emerging opportunities.
6. Automated trading and high-frequency execution
Algorithmic trading has been around for years, but AI refines it by making split-second decisions based on real-time analytics.
Example: AI-driven hedge funds employ deep learning models to execute trades with extreme precision, helping you maximize returns while carefully managing risk.
7. AI in investment committee meetings
Beyond direct financial analysis, AI can streamline your investment meetings by summarizing discussions, highlighting key decisions, and assigning follow-up actions. It can also look at past meeting data to identify trends or topics that need more attention.
Example: Platforms like adam.ai capture and summarize important points in real time, so you spend less time sifting through reports and more time acting on insights. Investment committees can improve productivity by reducing meeting fatigue with AI, ensuring discussions stay focused and outcome-driven.
What AI technologies are transforming financial data analysis and risk assessment?
Investment decisions have always relied on analyzing complex financial data, assessing risks, and predicting market movements. Traditionally, this was a slow, manual process prone to human error. AI is changing that. It’s not just making data analysis faster; it’s revealing patterns, identifying risks, and offering insights that humans might overlook. Here’s how:
1. Natural language processing
AI-powered natural language processing (NLP) scans and interprets vast amounts of text-based financial data: news articles, earnings call transcripts, analyst reports, and even social media. It doesn’t just read; it understands sentiment, context, and trends.
Example: Investment firms use NLP to analyze CEOs' tone in earnings calls. Subtle shifts in wording or hesitation might indicate financial instability before the numbers reflect it.
2. Machine learning
Machine learning (ML) models continuously learn from financial data, identifying trends that traditional models might miss. Unlike static formulas, ML algorithms adapt over time, refining predictions based on new data.
Example: Hedge funds use ML to forecast stock price movements by analyzing millions of historical and real-time data points, far beyond what human analysts can process.
3. Deep learning
Deep learning, a subset of machine learning, goes a step further. It mimics human thought processes but at a much greater scale, detecting complex relationships between variables. It’s particularly useful for fraud detection, credit risk assessment, and stress testing investment strategies.
Example: Banks use deep learning to detect potential loan defaults, analyzing everything from transaction histories to alternative data sources like online behavior.
4. AI-powered predictive analytics
Predictive analytics uses AI-driven models to anticipate market movements and economic shifts. By analyzing historical patterns, geopolitical events, and macroeconomic indicators, AI can suggest the best course of action for investments.
Example: AI models helped financial institutions predict market shocks during COVID-19 by analyzing real-time global data. Firms that relied on predictive analytics were able to adjust their portfolios faster.
5. Algorithmic trading
AI-driven trading algorithms execute transactions in fractions of a second, analyzing vast datasets to capitalize on opportunities before human traders can react. These models optimize buying and selling strategies by detecting short-term patterns and anomalies.
Example: High-frequency trading firms use AI to make split-second trade decisions based on real-time market signals, price fluctuations, and historical trends.
6. Generative AI
Generative AI is emerging as a key player in automated investment reporting and strategy formulation. It can summarize financial reports, create market insights, and even generate customized investment recommendations.
Example: Financial advisors are integrating AI chatbots that generate real-time reports and answer complex investment questions using historical data.
7. AI in meeting analytics
AI isn’t just analyzing markets; it’s transforming how investment committees discuss, decide, and document investment strategies. AI-powered meeting analytics can track decisions, extract action items, and ensure teams act on critical insights.
Example: adam.ai helps investment teams centralize discussions, track meeting outcomes, and ensure follow-ups happen, eliminating lost insights and inefficient decision-making.
Can AI accurately predict market trends and investor behavior?
AI has changed the way investors approach market forecasting. It’s fast, it’s data-driven, and it can process more information in a second than a human could in a lifetime. But does that mean AI can accurately predict the market? The answer: It can make highly informed forecasts, but it’s not a fortune teller. Let’s break it down.
AI’s strength in market trend prediction
AI isn’t about making wild guesses; it works by identifying patterns, correlations, and anomalies in massive datasets. It doesn’t "predict" in the way a human might; instead, it assigns probabilities to different market movements based on past behaviors and real-time inputs. Here’s where AI shines:
✅ Pattern recognition beyond human capability
AI can process decades of market data, identifying subtle indicators of a shift before they become obvious. It can compare current conditions to historical trends, flagging situations where similar market patterns led to a rally or downturn.
✅ Event-based predictions
While AI can’t predict the exact outcome of events like a Federal Reserve policy shift or a geopolitical crisis, it can analyze historical responses to similar scenarios and estimate how different asset classes might react.
✅ Real-time adjustment
Unlike static models, AI doesn’t rely only on past data. It constantly adjusts its predictions as new information, earnings reports, inflation rates, commodity prices, flows in.
Example: AI used by investment firms detected early signals of market volatility before the 2020 pandemic crash. It noticed supply chain disruptions, travel industry slowdowns, and shifts in bond yields: all signs that a major economic shock was on the way.
The challenge of predicting investor behavior
Markets aren’t just about numbers; they’re about people. And people? They’re unpredictable.
AI can track trends in investor sentiment, but human emotions, fear, greed, panic, still create situations that defy logic. Here’s where AI’s limitations become apparent:
❌ Unpredictable crowd behavior
AI can flag rising excitement or anxiety in the market, but it can’t always predict how far people will take it. Take the GameStop short squeeze; AI detected unusual social sentiment early on, but it didn’t predict the full scale of the retail trader movement.
❌ Black swan events
AI is great at recognizing patterns. But what about events with no precedent: pandemics, wars, or financial crises driven by unseen risks? AI can’t predict what it’s never seen before.
Example: In 2022, AI models tracking economic indicators failed to predict the speed of inflation surges, as they hadn’t encountered such rapid post-pandemic economic shifts in historical data.
So, is AI good enough?
Yes, AI is an invaluable tool for investment forecasting. It processes vast amounts of data, eliminates human bias, and spots market signals faster than any human. But it works best as an enhancement to human expertise, not a replacement.
Think of it this way: AI is like an advanced GPS for investors. It helps navigate complex market conditions, avoiding blind spots and roadblocks. But just like a GPS, it doesn’t control the road; only the driver does.
How does AI-driven forecasting improve investment strategy and portfolio management?
Investment decisions rely on a mix of data, timing, and adaptability. AI-driven forecasting gives investors an edge by making sense of massive datasets, detecting market patterns, and adjusting portfolio strategies based on real-time insights. Instead of reacting to changes after they happen, investors can adjust their positions proactively, reducing risk and capitalizing on emerging opportunities.
Market forecasting: AI processes information at a scale humans can’t match
Traditional forecasting methods depend on historical trends, economic reports, and analyst insights. AI adds another layer by continuously scanning global economic indicators, market sentiment, and asset performance to find patterns that hint at future movements.
Markets are complex, and no single factor drives change. AI can identify hidden correlations between seemingly unrelated events. When supply chain disruptions started affecting global trade, some investment firms adjusted early. Their AI models detected rising raw material costs, shifting trade patterns, and changes in corporate earnings calls: factors that traditional analysts reviewed in isolation.
Portfolio optimization: AI fine-tunes investment decisions in real time
A balanced portfolio today might be overexposed tomorrow. AI-driven forecasting helps investors adjust allocations based on live market conditions, not just historical trends. Unlike fixed asset allocation models, AI constantly evaluates risk levels, asset performance, and external economic shifts to recommend adjustments when needed.
Some firms use AI to monitor interest rate movements and cross-sector dependencies. When bond yields started rising, AI signaled the risk to growth stocks and suggested rotating into value sectors. This approach allows investors to maintain stability without manually rebalancing portfolios at set intervals.
Risk management: AI uncovers weak spots before they become liabilities
Risk isn’t always obvious. Some of the biggest losses come from factors investors don’t see coming: liquidity crunches, geopolitical instability, or a company’s deteriorating fundamentals hidden beneath strong quarterly reports. AI-driven models track these indicators as they develop, helping investors avoid high-risk positions before losses accumulate.
Hedge funds using AI for risk analysis detected trouble in overleveraged tech startups months before the downturn. By analyzing company debt levels, investor sentiment, and hiring trends, the AI flagged potential financial instability long before stock prices reflected it.
AI-driven insights improve investment committee decisions
Having access to better forecasts is only useful if investment teams act on them effectively. AI improves the way portfolio managers and committees use market insights by keeping data current, relevant, and structured for decision-making.
Firms that integrate AI into their investment reviews don’t waste time debating outdated projections. Instead, they focus on scenario testing, risk mitigation, and portfolio alignment with market conditions. AI-driven platforms also track past decisions, ensuring committees follow through on strategies rather than making fragmented adjustments.
Public-sector investment bodies are increasingly adopting AI for government committees and AI-driven documentation to ensure transparency, accuracy, and accountability in financial decision-making.
AI enhances investment strategies, but human expertise remains essential
Predicting the market with absolute certainty isn’t possible. What AI does well is reduce uncertainty by identifying trends early, adjusting risk exposure, and refining portfolio strategies based on real-time data. The firms using AI-driven forecasting effectively are those that blend its insights with human expertise, ensuring that every investment decision is backed by both data and judgment.
How can AI meeting analytics improve investment discussions and decision-making?
Investment committees handle complex discussions where every decision can impact portfolios, risk exposure, and long-term returns. The challenge is not just making the right calls but ensuring that discussions are well-informed, data-driven, and consistently followed through. AI meeting analytics help by capturing insights, tracking decisions, and providing real-time data that enhances the quality of investment discussions.
Data-backed decision-making: AI ensures meetings are based on current insights
Investment discussions often rely on reports prepared days or weeks in advance. AI eliminates this lag by integrating live market data, portfolio performance metrics, and risk assessments directly into the discussion. Instead of debating outdated numbers, teams can work with real-time analytics and up-to-date financial models to make better investment calls.
Firms using AI-driven analytics have seen a shift in how investment committees operate. Meetings are no longer focused on reconfirming old projections but on adjusting strategies based on fresh, relevant data. This approach leads to quicker, more decisive action when market conditions change.
Tracking investment decisions: AI ensures accountability and follow-through
A common issue in investment meetings is decision fatigue. Discussions may result in strong recommendations, but without a clear system to track follow-ups, key actions can get lost. AI analytics automatically log investment decisions, track commitments, and send reminders to ensure that resolutions do not get buried in meeting notes.
Organizations leveraging AI-powered meeting management solutions can significantly improve the ROI of reduced meeting time by optimizing decision-making processes and eliminating unnecessary discussions.
For portfolio managers, this means greater consistency in executing strategies and fewer instances where committee discussions do not translate into real-world actions. Investment teams using AI meeting analytics report better alignment between strategy and execution because decisions are recorded, monitored, and acted upon in a structured way.
Sentiment and engagement analysis: AI improves the quality of discussions
Investment meetings involve multiple voices, each bringing different perspectives on risk, allocation, and opportunities. AI can analyze meeting engagement levels, sentiment shifts, and discussion patterns to ensure that every viewpoint is considered. If certain topics dominate discussions while critical risks get overlooked, AI can flag these imbalances.
Firms using AI-powered sentiment analysis have identified trends in meeting dynamics that affect decision-making. For example, AI can highlight when certain asset classes receive disproportionate attention while risk discussions are cut short. This helps committees adjust their approach to ensure that investment decisions remain balanced and well-informed.
Reducing cognitive overload: AI automates meeting documentation
Committee members deal with large volumes of financial reports, risk assessments, and economic forecasts. AI meeting analytics automate the process of summarizing discussions, extracting key insights, and organizing decisions into structured action points. Instead of sifting through dense meeting notes, teams receive clear, concise summaries that highlight next steps.
AI enhances investment discussions by using machine learning in meeting preparation to analyze previous meeting insights, suggest relevant agenda items, and structure discussions more effectively.
For firms managing multiple portfolios, this reduces administrative overhead and allows teams to focus on evaluating market conditions rather than managing documentation. AI-generated reports also ensure that key takeaways are accessible to stakeholders who could not attend the meeting, improving transparency across investment teams.
AI strengthens investment committees without replacing human expertise
AI does not make investment decisions, but it ensures that discussions are more focused, data-driven, and actionable. It eliminates inefficiencies, enhances engagement, and keeps decision-making aligned with real-time financial conditions. Investment committees using AI meeting analytics are not just improving discussions. They are ensuring that every meeting moves strategies forward with greater precision and accountability.
How can organizations integrate AI without replacing human expertise?
AI is reshaping investment decision-making, but it works best as a complement to human expertise rather than a replacement. Organizations that integrate AI successfully use it to enhance analysis, automate repetitive tasks, and provide real-time insights while keeping human oversight at the center of strategic decision-making.
Enhancing, not replacing: AI supports human judgment
AI processes vast amounts of financial data quickly, but investment decisions require more than just pattern recognition. Human analysts bring intuition, experience, and the ability to interpret context—factors AI cannot fully replicate. Instead of relying on AI to make final calls, firms can use it to generate forecasts, detect anomalies, and provide scenario analyses that help professionals make more informed, strategic decisions.
Investment committees, for example, use AI-driven analytics to assess risk and market conditions, but human experts still determine when and how to act based on macroeconomic trends, geopolitical risks, and industry knowledge.
Blending automation with oversight: AI reduces workload without eliminating critical thinking
AI can handle routine processes such as data aggregation, report generation, and portfolio rebalancing, freeing investment professionals to focus on higher-level strategy. By automating repetitive tasks, firms reduce human error and improve efficiency while ensuring that final decisions remain in human hands.
For example, asset managers use AI-driven tools to monitor portfolio performance and risk exposure in real time. The AI flags areas of concern, but it is up to the portfolio manager to decide whether to rebalance assets, hedge risks, or adjust allocations.
Keeping AI in check: Transparent models and explainable decisions
One challenge with AI integration is that some models function as black boxes, making decisions based on complex algorithms without clear explanations. Organizations must prioritize explainable AI, ensuring that financial professionals understand how models generate insights.
Regulatory bodies require financial institutions to justify AI-driven investment decisions, so firms must adopt AI tools that provide clear reasoning and transparent methodologies. Human oversight ensures that AI does not introduce bias, make flawed assumptions, or overlook qualitative factors.
Training and collaboration: Building AI fluency within investment teams
Successful AI integration requires investment professionals to understand how to use AI effectively. Organizations should train teams on AI capabilities, limitations, and best practices. Instead of viewing AI as a replacement, firms should encourage collaboration between human analysts and AI-driven insights.
Some investment firms have created hybrid decision-making models, where AI provides initial forecasts and scenario analyses, but investment teams evaluate and refine the recommendations before taking action. This approach ensures that AI remains a tool rather than a decision-maker.
What are some real-world examples of AI-powered investment decision-making?
AI is not just a theoretical advantage in investment management. It is already being used by financial institutions, hedge funds, and asset managers to improve market forecasting, risk assessment, and portfolio optimization. Here are some real-world examples of how AI is shaping investment decision-making today.
Hedge funds using AI-driven trading strategies
Several hedge funds rely on AI to execute trades based on real-time market analysis. AI algorithms track financial data, investor sentiment, and macroeconomic indicators to identify profitable trading opportunities faster than human analysts.
One well-known example is Bridgewater Associates, which uses machine learning models to analyze economic trends and automate investment decisions. By processing vast amounts of data, AI helps the fund adapt its strategies dynamically in response to market conditions.
AI-powered ETFs and portfolio management
Exchange-traded funds (ETFs) are increasingly incorporating AI to manage portfolios. The Amplify AI Powered Equity ETF (AIEQ) uses IBM Watson’s AI technology to select stocks based on fundamental and technical factors. The AI continuously analyzes news reports, earnings data, and sentiment indicators to adjust the fund’s holdings.
This approach allows AI-driven ETFs to react quickly to changing market conditions, helping investors optimize performance without relying on traditional human fund managers.
AI in investment banking and risk management
Large financial institutions use AI to assess risk and detect market anomalies. JPMorgan Chase developed an AI-powered system called LOXM, which optimizes trade execution by analyzing historical transaction data and market liquidity. LOXM ensures that large trades are executed at the best possible price while minimizing market impact.
Similarly, Goldman Sachs uses AI to improve risk modeling and credit assessments. The firm employs machine learning algorithms to analyze creditworthiness, predict loan defaults, and automate parts of the underwriting process.
Retail trading platforms integrating AI for investors
AI is also making advanced investment strategies accessible to retail investors. Platforms like Betterment and Wealthfront use AI-driven robo-advisors to create personalized investment portfolios based on an individual’s financial goals, risk tolerance, and market trends.
These platforms continuously monitor market conditions and automatically rebalance portfolios, ensuring that investments remain aligned with user preferences and risk exposure.
AI-powered sentiment analysis for market predictions
Financial firms use AI to analyze investor sentiment from news sources, earnings call transcripts, and social media. AI models at firms like BlackRock process vast amounts of unstructured text data to gauge market sentiment and adjust investment strategies accordingly.
For example, during major economic events such as Federal Reserve policy announcements, AI-powered sentiment analysis helps asset managers predict how investors might react and adjust portfolios in advance.
AI improving investment meetings and strategic planning
Beyond direct trading, AI is also transforming how investment committees and portfolio managers conduct meetings and make strategic decisions. AI meeting analytics track discussions, extract key insights, and ensure that follow-up actions align with financial strategies.
Firms using AI-powered investment meetings report improved decision-making efficiency, as AI helps consolidate data, highlight risk factors, and streamline strategic discussions.
How does adam.ai empower investment teams with AI-driven meeting insights?
Investment teams need structured discussions, real-time data sharing, and clear follow-ups to make informed financial decisions. Adam.ai enhances investment meetings by providing AI-driven features that ensure every discussion is well-organized, data-driven, and actionable. Here's how
- Agenda management: Investment committees can structure meetings by setting clear discussion points, allocating time for critical topics, and ensuring that financial strategies remain the focus.
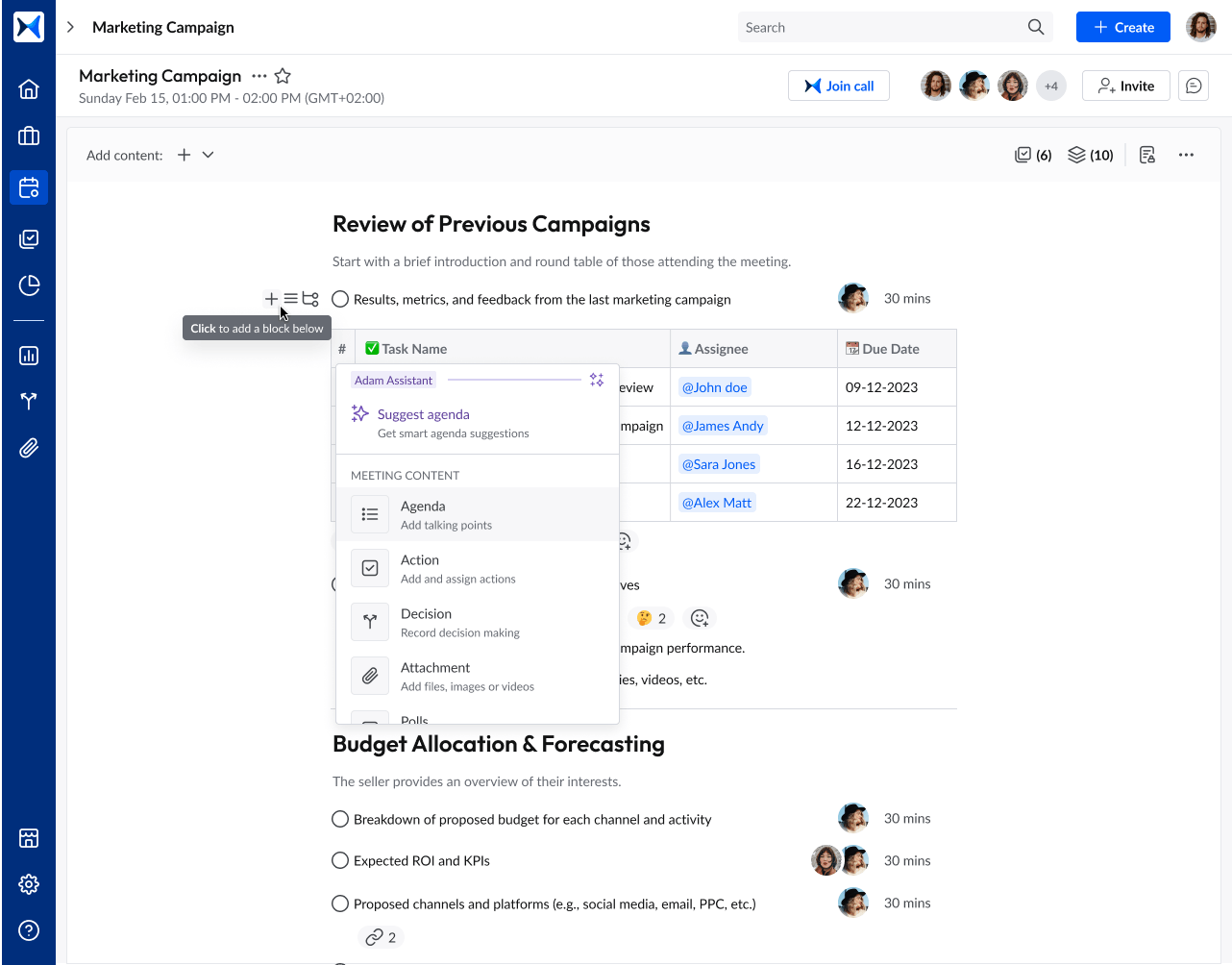
- Real-time content collaboration: Teams can share and edit reports, financial models, and risk assessments during meetings, ensuring that investment decisions are based on the latest data.
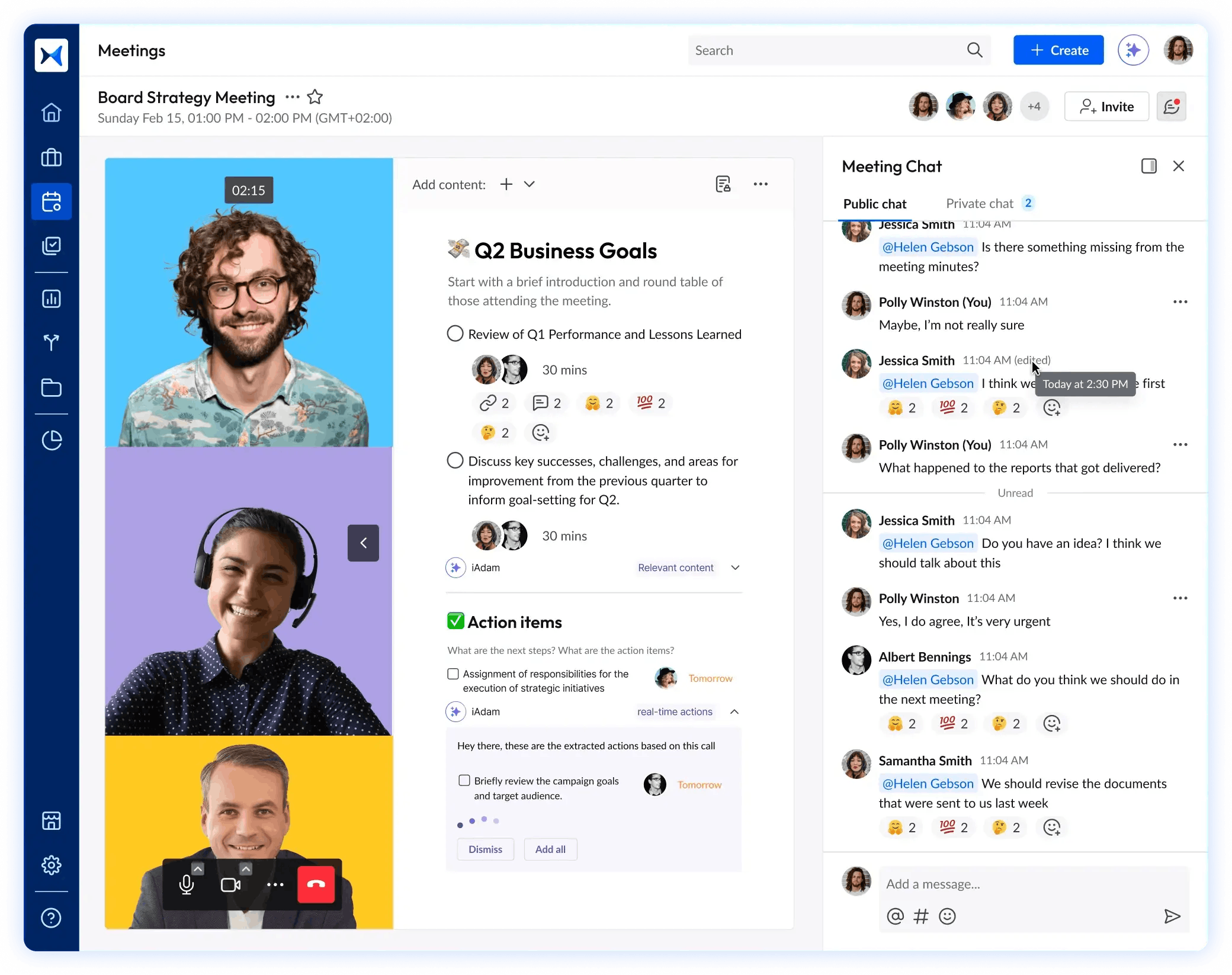
- Action tracking: Every decision—whether adjusting asset allocations, mitigating risks, or approving new investments—is logged and assigned to the right team members for follow-up.
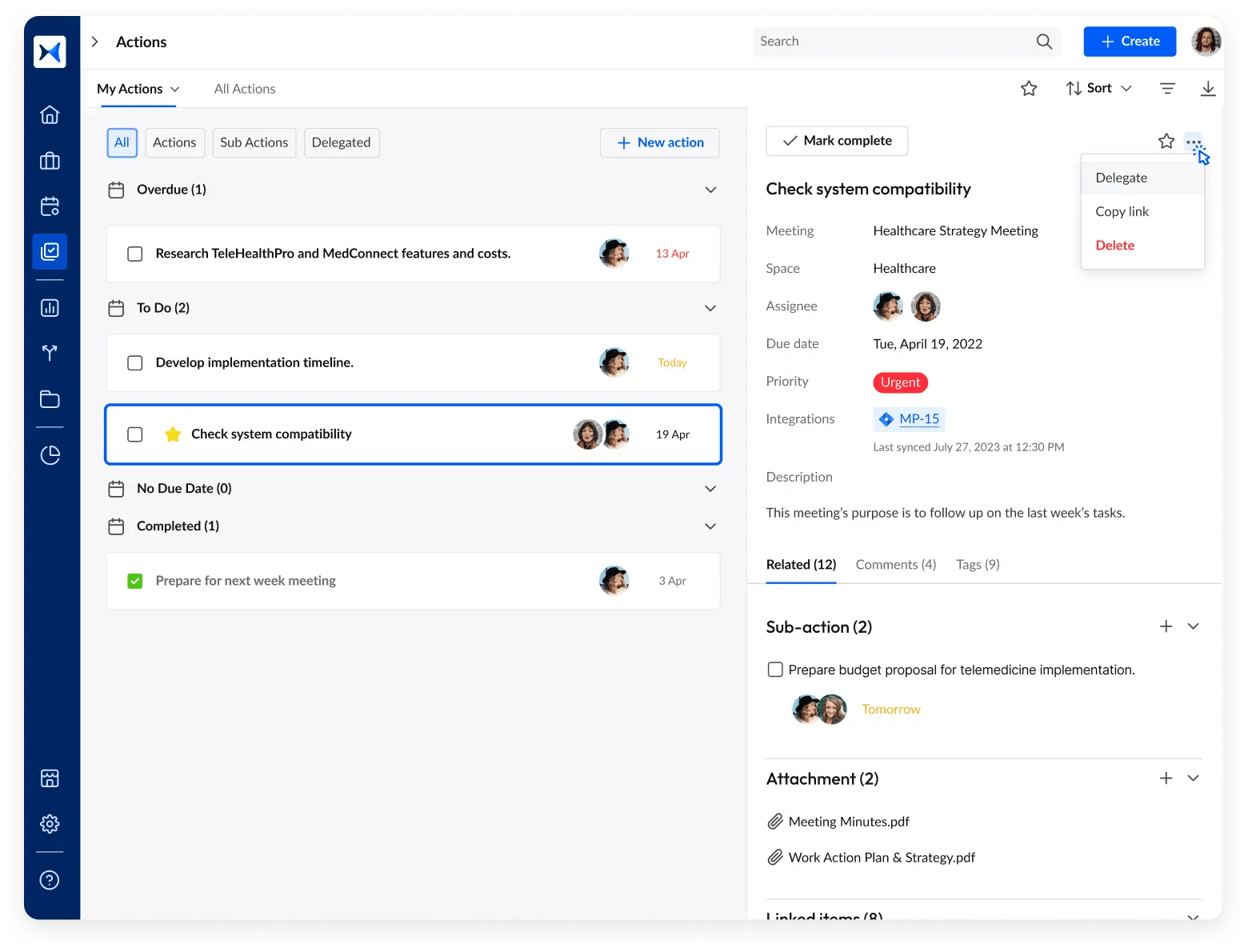
- Meeting minutes: AI-generated summaries capture key discussions, portfolio adjustments, and strategy changes, ensuring that investment teams can reference past decisions with full context.
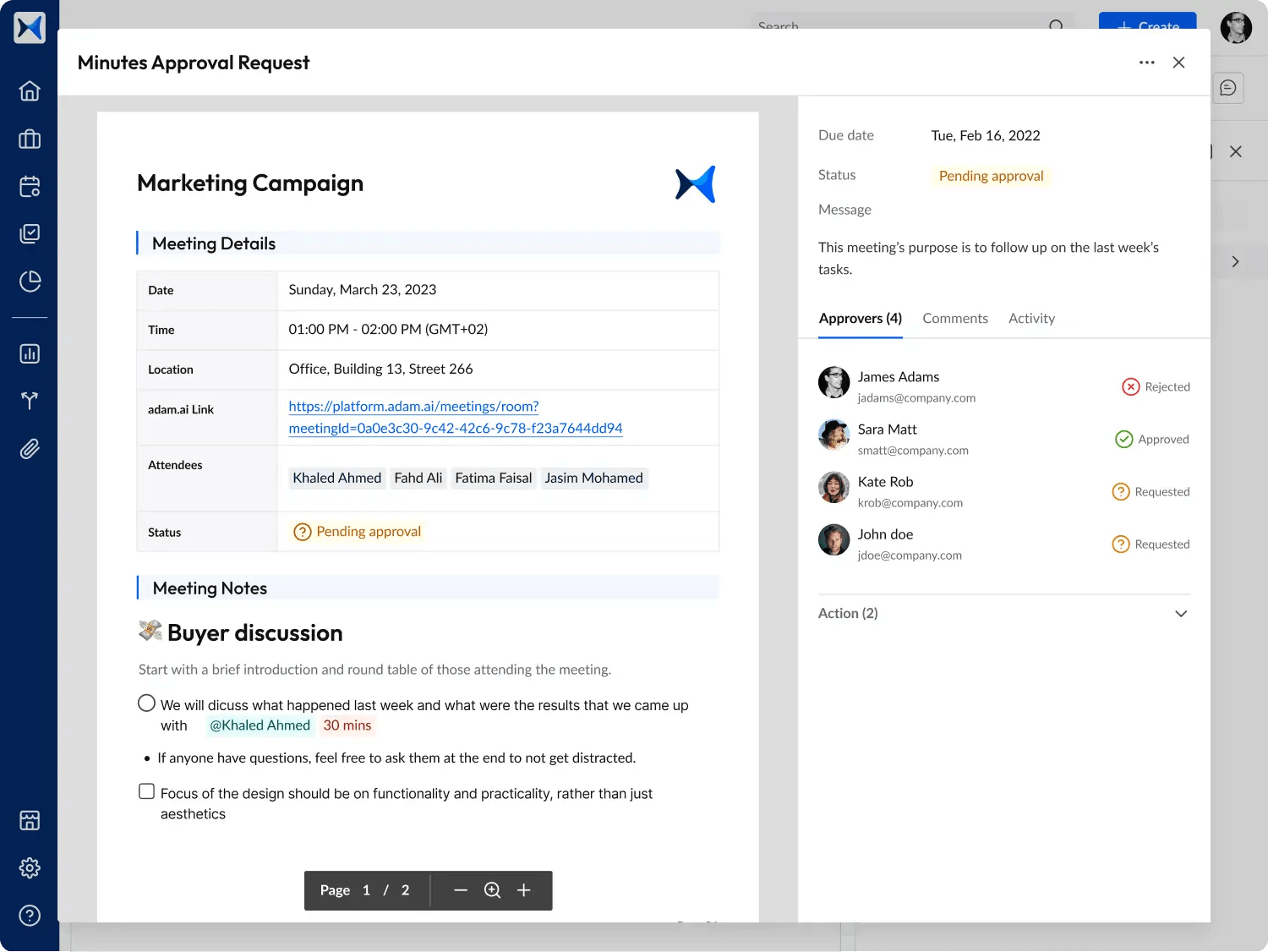
- Multi-space management: Firms managing multiple funds, portfolios, or investment strategies can organize meetings across different spaces, keeping decisions aligned with specific financial goals.
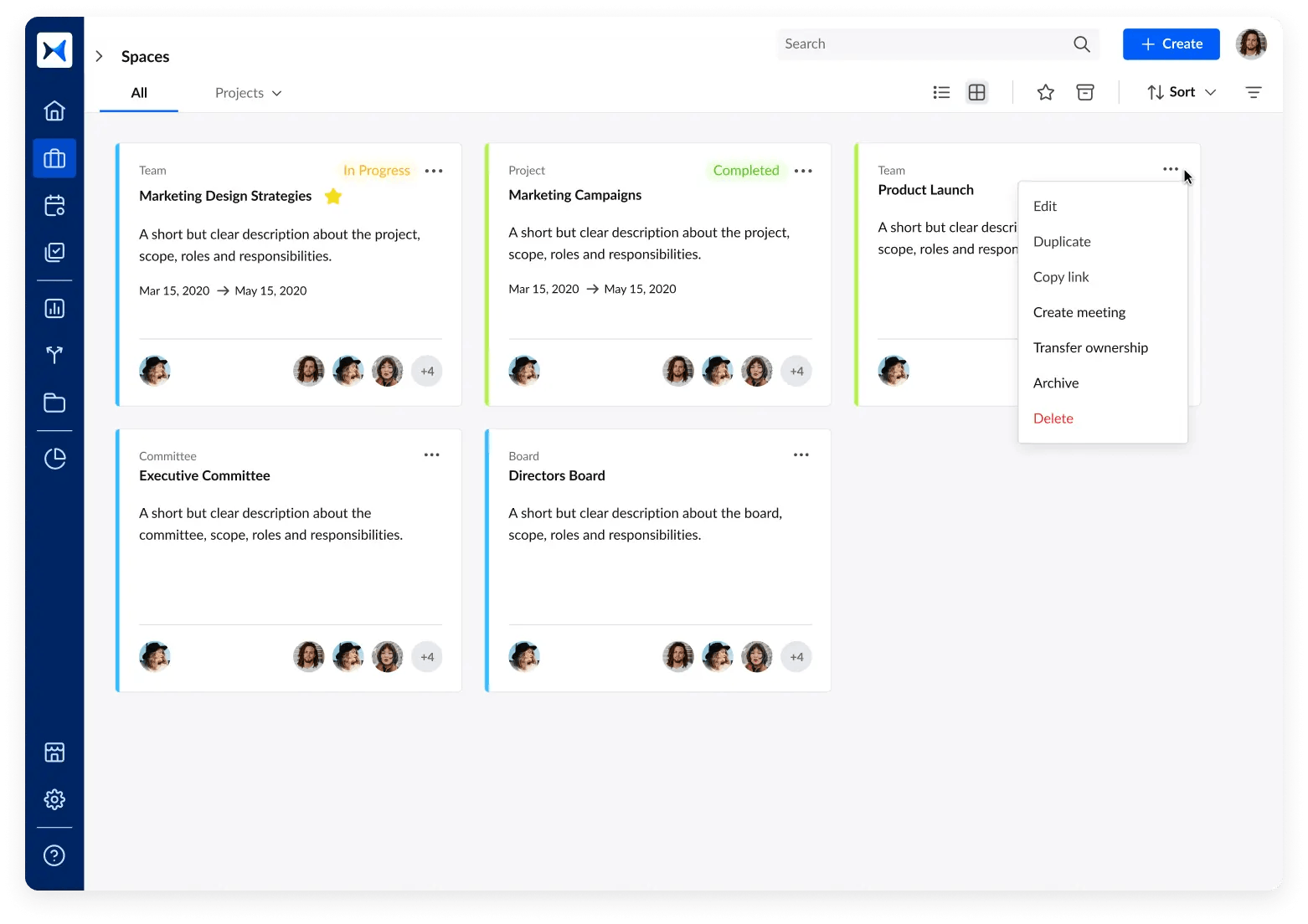
- Analytical dashboards: Teams gain access to real-time insights from past meetings, allowing them to track trends in investment decisions, risk assessments, and portfolio adjustments over time.
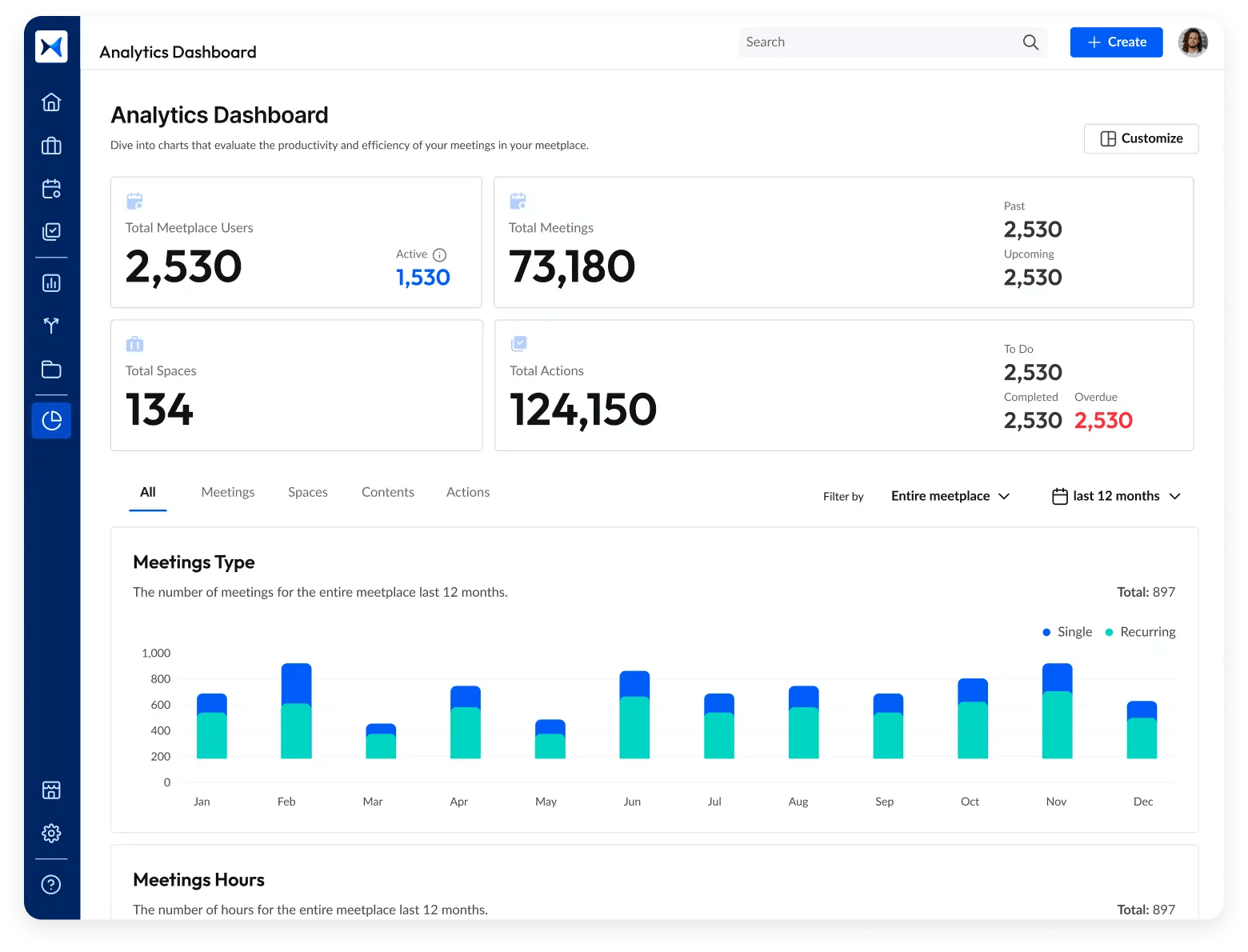
Transform how you conduct critical meetings—From meticulous preparation to effective execution and insightful follow-up, adam.ai integrates comprehensive analytics, full customization, and intuitive interfaces with powerful meeting management tools.
Easy onboarding. Enterprise-grade security. 24/7 dedicated support.
The bottom line
Investment meetings should not end with vague discussions and forgotten action points. Modern tools ensure that every decision is backed by structured agendas, real-time collaboration, and data-driven insights.
And while there may be multiple solutions available, here is why adam.ai is the meeting management software platform you can trust:
- adam.ai is one of Atlassian Ventures' portfolio companies.
- In the meeting management software category on G2, adam.ai has been ranked a leader and a high performer for successive quarters in the past years.
- adam.ai has been included in the Forrester Report in the AI-enabled meeting technology landscape.
- adam.ai is trusted and used by powerful teams and organizations worldwide for all types of critical meetings, like board, committee, project management, and business development meetings.
- And most importantly, adam.ai integrates with your existing workflow, is SOC2 compliant, provides dedicated support and success, and has a free trial option.
Subscribe to adam.ai blog
Stay ahead with the latest insights—get our newest blog posts, tips, and updates sent straight to your inbox.