December 11, 2024 · 13 min read
Integrating Machine Learning for Proactive Meeting Preparation
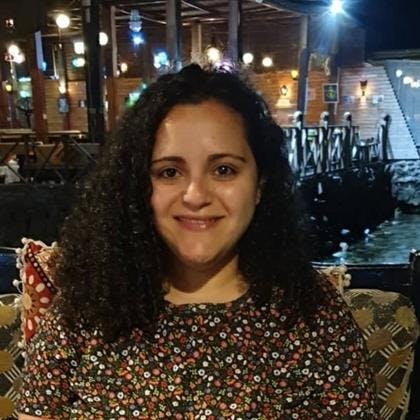
Shaimaa Badawi
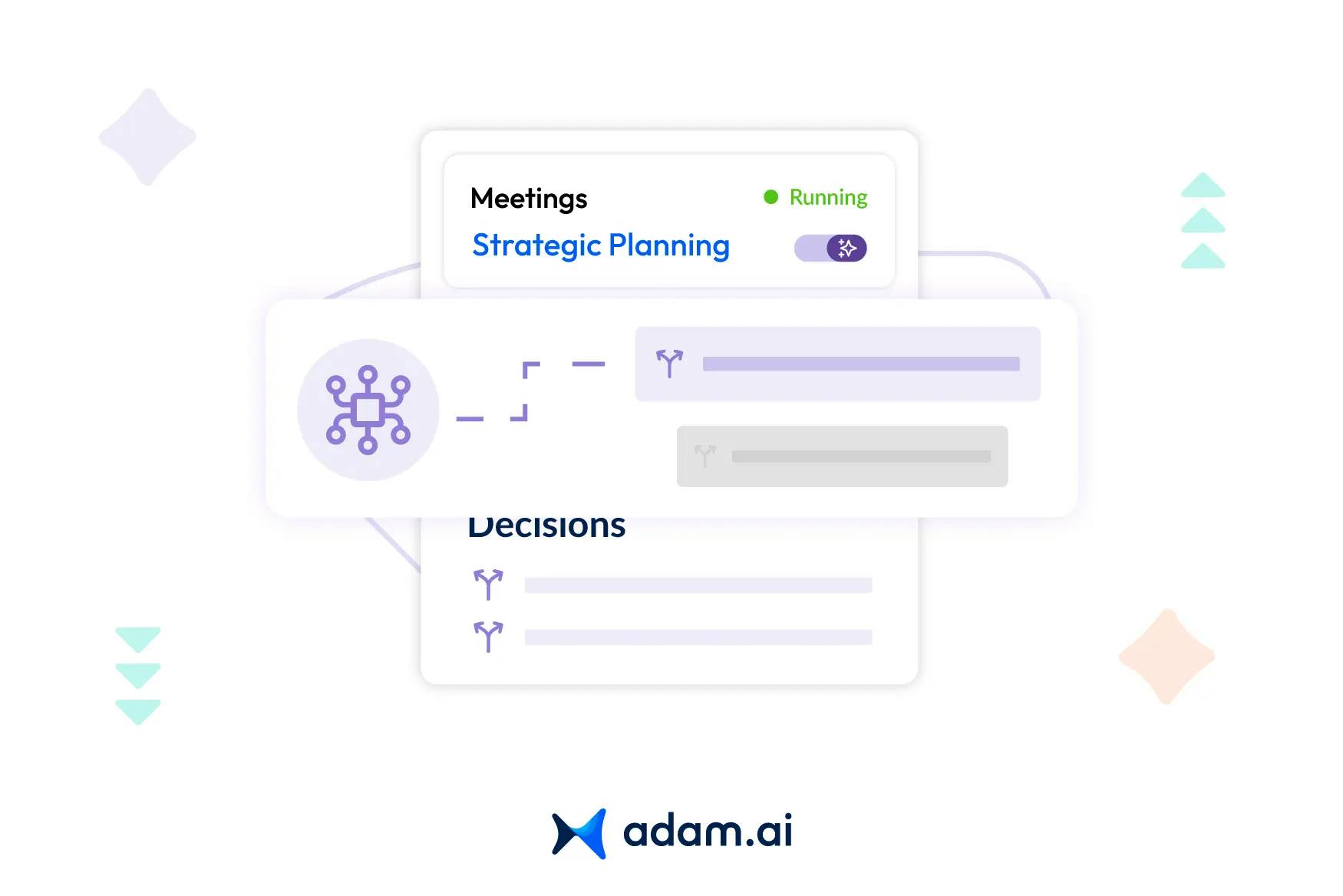
Proactive meeting preparation is essential for driving efficiency and ensuring productive outcomes. With the rise of machine learning, organizations now have powerful tools to automate and streamline the preparation process. In this article, we explore how machine learning transforms meeting workflows, from creating personalized agendas to automating follow-ups, and how these advancements enhance collaboration and decision-making.
What is machine learning, and how does it enable smarter decision-making?
Machine learning (ML) is a subset of artificial intelligence (AI) that empowers machines to learn from data and improve their performance without explicit programming. By analyzing patterns, relationships, and trends within data, ML algorithms can make predictions, classify information, and provide actionable insights, enabling smarter and faster decision-making.
How machine learning enables smarter decision-making
- Pattern recognition and insights
ML excels at identifying patterns and trends in vast datasets that may be too complex for human analysis. For example, it can detect customer behavior trends, predict equipment failures, or identify anomalies in financial transactions. - Real-time analysis
With its ability to process data in real-time, ML supports dynamic decision-making. This is particularly valuable in scenarios like stock market trading, fraud detection, or optimizing workflows during live operations. - Predictive analytics
ML algorithms use historical data to forecast future outcomes, helping organizations anticipate trends, mitigate risks, and seize opportunities. Predictive analytics is widely used in industries like healthcare, finance, and logistics. - Automation and efficiency
By automating routine and repetitive tasks, ML frees up human resources for more strategic activities. For example, it can streamline processes such as email categorization, inventory management, or meeting agenda creation. - Personalized recommendations
ML enables personalization by tailoring recommendations based on individual preferences or past behavior. This technology drives applications ranging from streaming services to e-commerce platforms. - Adaptability and continuous improvement
Machine learning models evolve with new data, allowing them to refine their accuracy and effectiveness over time. This adaptability ensures that decision-making remains relevant in dynamic environments.
Why is proactive preparation crucial for successful meetings?
Proactive preparation is essential for successful meetings because it ensures that discussions are focused, productive, and aligned with organizational goals. By anticipating needs and addressing potential challenges ahead of time, proactive preparation creates a foundation for informed decision-making and meaningful outcomes.
Why proactive preparation is crucial
- Clarity of objectives
Proactive preparation helps define clear objectives for the meeting, ensuring that all participants understand the purpose and desired outcomes. This clarity minimizes confusion and keeps discussions on track. - Efficient use of time
Preparing in advance ensures that agendas are well-structured, materials are distributed, and logistics are in place. This prevents time from being wasted on last-minute arrangements or unproductive conversations. - Enhanced collaboration
When participants come prepared, they can contribute valuable insights and engage in meaningful discussions. This fosters a collaborative environment where diverse perspectives are shared and respected. - Data-driven decisions
Proactive preparation involves gathering relevant data and insights before the meeting. This allows participants to base their decisions on accurate, up-to-date information, reducing guesswork and enhancing the quality of outcomes. - Reduced stress and conflict
Addressing potential challenges or questions in advance minimizes disruptions during the meeting. Participants are more likely to feel confident and aligned, reducing stress and the likelihood of conflict. - Actionable follow-ups
A well-prepared meeting sets the stage for clear action items and responsibilities. This ensures that decisions made during the meeting are effectively implemented and monitored.
How can machine learning enhance the efficiency of meeting preparation?
Machine learning enhances the efficiency of meeting preparation by automating repetitive tasks, analyzing complex data, and providing personalized insights. By leveraging ML algorithms, organizations can save time, improve accuracy, and create well-structured meetings tailored to specific goals and participants.
How ML enhances meeting preparation
- Automating agenda creation
ML can analyze past meeting data and suggest agenda items, prioritize topics, and allocate time slots based on previous discussions. This ensures that meetings are structured and focused on critical issues. - Identifying relevant documents
By scanning organizational databases, ML algorithms can identify and recommend documents, reports, or presentations relevant to the meeting’s objectives. This reduces the manual effort required to gather materials. - Analyzing attendee needs
ML can assess participants’ roles, expertise, and past contributions to suggest topics or tailor discussions to their strengths, ensuring a more engaging and productive meeting. - Predicting potential discussion points
Predictive analytics can highlight likely discussion points based on trends or unresolved issues from previous meetings. This allows organizers to address critical topics proactively. - Streamlining scheduling
ML-powered tools can identify optimal meeting times by analyzing participants’ calendars and availability, minimizing scheduling conflicts and ensuring maximum attendance. - Enhancing preparation with insights
ML can analyze data from various sources to generate insights or recommendations, enabling participants to come to the meeting with a clearer understanding of the context and goals.
Example in practice
Imagine a project kickoff meeting where ML algorithms analyze the project scope, previous project data, and team roles. The system generates an agenda, identifies necessary resources, and highlights key risks to be discussed, enabling a productive and efficient start to the project.
What role does data quality play in effective machine learning for meetings?
Data quality plays a pivotal role in effective ML for meetings, as it directly impacts the accuracy, relevance, and usefulness of the outcomes generated by ML algorithms. High-quality data ensures that ML models can reliably analyze patterns, make predictions, and provide actionable insights, enabling smarter and more efficient meeting preparation.
Key roles of data quality
- Accuracy of recommendations
Clean and accurate data ensures that ML-generated agendas, action items, and insights are precise and relevant to the meeting's goals. Poor-quality data can lead to irrelevant or incorrect suggestions, undermining the effectiveness of the preparation process. - Relevance to meeting context
High-quality data allows ML algorithms to focus on information that is directly applicable to the meeting’s objectives. This ensures that recommendations align with the needs and priorities of participants. - Reduction of bias
Diverse and representative datasets help minimize bias in ML models. For meetings, this means that the insights generated are inclusive and consider varied perspectives, fostering balanced and productive discussions. - Improved predictive accuracy
Quality data enhances the predictive capabilities of ML algorithms, enabling accurate forecasting of discussion points, potential conflicts, or future action items based on past meeting trends. - Efficiency in automation
Structured and well-maintained data enables ML tools to automate processes like agenda creation, resource identification, and scheduling seamlessly, reducing the manual effort required for preparation. - Trust in ML-driven insights
High-quality data builds confidence in the recommendations and outputs provided by ML systems. Participants are more likely to trust and adopt ML-driven insights when they are consistently accurate and reliable.
Example in practice
Consider a meeting preparation tool that relies on historical data to suggest agenda items and assign responsibilities. If the underlying data includes outdated or incomplete records, the suggestions may be irrelevant or impractical. In contrast, high-quality data enables the tool to provide actionable and meaningful insights, streamlining preparation.
How do adaptive algorithms personalize meeting agendas for different teams?
Adaptive algorithms personalize meeting agendas for different teams by analyzing data patterns, roles, past interactions, and team-specific needs. By leveraging ML techniques, these algorithms dynamically create agendas that align with the unique goals, preferences, and work styles of each team.
How adaptive algorithms personalize meeting agendas
- Analyzing team roles and responsibilities
Adaptive algorithms assess the roles and expertise of team members to tailor agenda topics. For instance, technical discussions might be prioritized for engineering teams, while strategic initiatives may be highlighted for leadership teams. - Learning from past meetings
Algorithms review historical meeting data to identify recurring topics, unresolved issues, or successful practices. This ensures the new agenda reflects ongoing priorities and avoids repetitive discussions. - Considering team objectives
By aligning with the team’s specific goals, such as project milestones, sales targets, or process improvements, adaptive algorithms ensure the agenda is relevant and actionable. - Incorporating participant preferences
Algorithms analyze participant feedback or engagement levels from previous meetings to adjust the focus or format. For example, if team members prefer concise updates, the agenda can reflect this preference. - Real-time data integration
Adaptive algorithms pull in real-time data from project management tools, task trackers, or CRM systems to highlight critical updates or action items, ensuring the agenda remains timely and informed. - Dynamic adjustment for team composition
When team composition changes, for instance, when external stakeholders join, a personalized agenda includes topics relevant to the new participants, fostering inclusivity and productivity.
Example in practice
For a cross-departmental project update, an adaptive algorithm tailors the agenda by prioritizing technical progress for developers, budget updates for finance, and milestone tracking for project managers. This ensures all participants receive the information most relevant to their roles.
What are some real-world examples of machine learning-driven proactive planning?
Machine learning-driven proactive planning is transforming industries by enabling organizations to anticipate needs, optimize workflows, and make data-driven decisions. Here are some real-world examples that showcase its applications across various sectors:
1. Predictive maintenance in manufacturing
- How it works: ML models analyze sensor data from machinery to detect patterns indicating potential failures.
- Proactive planning: Maintenance schedules are optimized to prevent breakdowns, reducing downtime and saving costs.
- Impact: Companies like Siemens and GE use predictive maintenance to enhance operational efficiency and extend equipment lifespan.
2. Dynamic inventory management in retail
- How it works: ML analyzes sales trends, customer preferences, and supply chain data to forecast demand.
- Proactive planning: Retailers can stock products based on predicted demand spikes, preventing overstock or shortages.
- Impact: Walmart and Amazon leverage such systems to streamline inventory and enhance customer satisfaction.
3. Personalized learning paths in education
- How it works: Adaptive learning systems use ML to assess student performance and recommend tailored content.
- Proactive planning: Educators receive insights to adjust teaching strategies, and students are guided through customized learning pathways.
- Impact: Platforms like Duolingo and Coursera improve engagement and learning outcomes.
4. Fraud detection in banking
- How it works: ML algorithms analyze transaction patterns to identify anomalies or suspicious activities.
- Proactive planning: Alerts are generated before fraudulent transactions occur, allowing banks to take preventive measures.
- Impact: Financial institutions like JP Morgan and PayPal use such systems to enhance security and trust.
5. Event planning in enterprises
- How it works: ML tools predict attendee preferences, optimize schedules, and suggest resources for meetings or conferences.
- Proactive planning: Organizers tailor events to audience needs, ensuring better engagement and outcomes.
- Impact: Corporate platforms like Bizzabo and Cvent employ these systems for event success.
6. Proactive meeting preparation
- How it works: ML analyzes historical meeting data, participant roles, and project updates to suggest agenda items and resources.
- Proactive planning: Teams enter meetings with a clear focus, ensuring time is spent on strategic discussions rather than ad-hoc organization.
- Impact: Platforms like adam.ai leverage ML to streamline meeting preparation and improve decision-making efficiency.
7. Supply chain optimization in logistics
- How it works: ML predicts delivery times, identifies risks in transportation routes, and suggests alternative paths.
- Proactive planning: Companies optimize logistics, reducing delays and costs while improving customer satisfaction.
- Impact: DHL and FedEx use such systems to ensure timely and efficient deliveries.
What challenges can organizations face when adopting machine learning for meetings?
Organizations face several challenges when adopting ML for meeting management. These challenges stem from technical, organizational, and cultural factors, which can hinder the effective integration of ML into workflows.
Challenges organizations face
1. Data quality and availability
- Issue: ML algorithms rely on high-quality, structured, and comprehensive data. Inconsistent, incomplete, or outdated meeting records can lead to inaccurate insights or predictions.
- Impact: Poor data quality reduces the reliability of automated agenda creation, action tracking, and predictive analytics.
2. Integration with existing tools
- Issue: Many organizations use diverse tools for meeting management, such as calendars, project management software, and communication platforms. Integrating ML systems with these tools can be complex.
- Impact: Fragmented workflows can reduce the effectiveness of ML-driven meeting solutions.
3. Resistance to change
- Issue: Employees may resist adopting ML-driven tools due to a lack of familiarity or fear of being replaced.
- Impact: Low adoption rates can prevent organizations from realizing the full potential of ML in meetings.
4. Ethical and privacy concerns
- Issue: Meeting data often contains sensitive or confidential information. Implementing ML systems raises concerns about data security, access control, and compliance with privacy regulations.
- Impact: Failure to address these concerns can result in a lack of trust and potential legal issues.
5. Skill gaps and training needs
- Issue: Organizations may lack the technical expertise needed to implement, maintain, and optimize ML systems.
- Impact: This can lead to reliance on external vendors or a steep learning curve for internal teams.
6. High initial costs
- Issue: Implementing ML systems involves upfront investments in software, infrastructure, and training.
- Impact: Smaller organizations or those with limited budgets may find it challenging to justify the expense.
7. Adapting to evolving needs
- Issue: Organizational goals and workflows evolve over time, and ML systems need to adapt to these changes.
- Impact: Static or poorly maintained models may become obsolete, reducing their relevance and utility.
Overcoming challenges
- Improve data quality: Implement processes for cleaning, validating, and organizing meeting data.
- Foster integration: Use platforms that seamlessly integrate with existing tools and workflows.
- Build trust: Educate employees on the benefits of ML and address concerns about job displacement.
- Ensure privacy compliance: Adopt robust security measures and comply with relevant data protection regulations.
- Invest in training: Upskill employees to work effectively with ML tools and systems.
Why is integrating machine learning a strategic advantage for enterprises?
Integrating machine learning (ML) offers a strategic advantage for enterprises by enabling smarter decision-making, improving efficiency, and fostering innovation. By harnessing the power of data, ML transforms operations and drives competitive edge across all levels of an organization.
Key strategic advantages
1. Enhanced decision-making
- Impact: ML analyzes large volumes of data to uncover patterns and trends, enabling data-driven decisions. Enterprises can make more accurate predictions and reduce the risks associated with uncertainty.
- Example: Predictive analytics helps forecast market trends, allowing businesses to align strategies with future demands.
2. Automation and efficiency
- Impact: ML automates repetitive tasks, such as data processing or scheduling, freeing up employees to focus on high-value activities. This improves productivity and reduces operational costs.
- Example: In meeting preparation, ML automates agenda creation and action tracking, streamlining workflows.
3. Personalization and adaptability
- Impact: ML tailors solutions to specific enterprise needs, providing personalized insights for teams or projects. It continuously learns and adapts to changing business requirements, ensuring relevance over time.
- Example: Adaptive algorithms create customized agendas based on team roles and objectives, improving meeting outcomes.
4. Proactive problem-solving
- Impact: ML enables enterprises to anticipate challenges and address them before they escalate. This proactive approach minimizes disruptions and enhances resilience.
- Example: Predictive maintenance powered by ML prevents equipment failures, reducing downtime and costs.
5. Scalability across functions
- Impact: ML solutions scale seamlessly with business growth, integrating into various functions such as operations, marketing, and finance.
- Example: ML-driven analytics optimize resource allocation across departments, ensuring efficient use of enterprise assets.
6. Driving innovation
- Impact: ML fosters innovation by enabling new business models, products, and services. Its ability to process and analyze unstructured data opens up opportunities for creative problem-solving.
- Example: Enterprises use ML to analyze customer feedback and innovate based on evolving market demands.
7. Competitive edge
- Impact: Organizations that adopt ML early can outperform competitors by leveraging its capabilities for speed, precision, and adaptability.
- Example: ML-powered recommendation systems improve customer engagement, boosting sales and loyalty.
How does adam.ai leverage machine learning for proactive meeting preparation?
adam.ai leverages machine learning to enhance proactive meeting preparation by integrating its core features into intelligent workflows. Here’s how these features align with the topic:
- Agenda management: adam.ai uses machine learning to analyze past meeting data and suggest agenda items tailored to specific goals and participants. This ensures that every meeting starts with a clear, relevant, and actionable agenda.
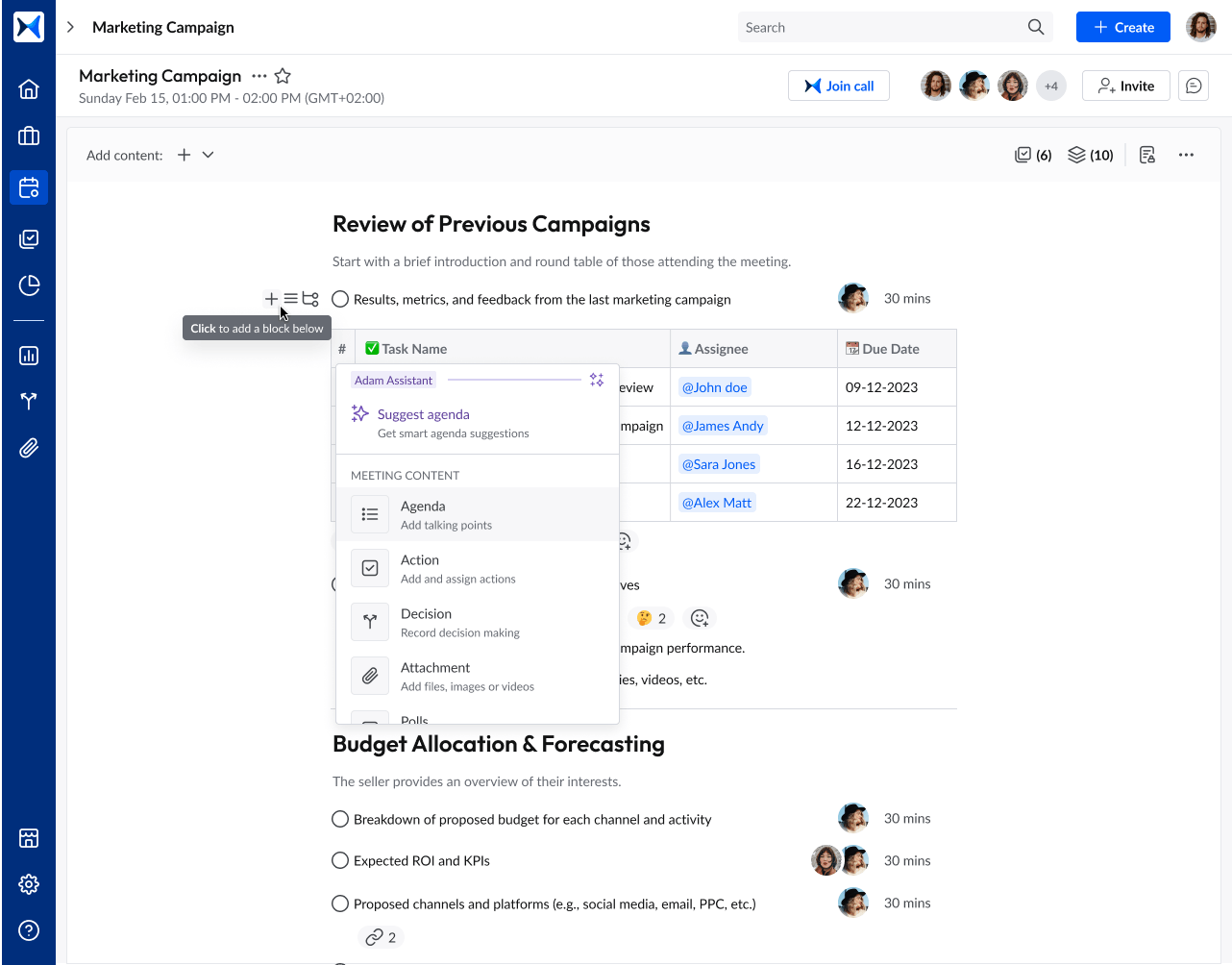
- Content collaboration: By analyzing organizational data, adam.ai recommends relevant documents or links, making collaboration seamless. This feature allows participants to review necessary materials in advance, ensuring meaningful contributions during the meeting.
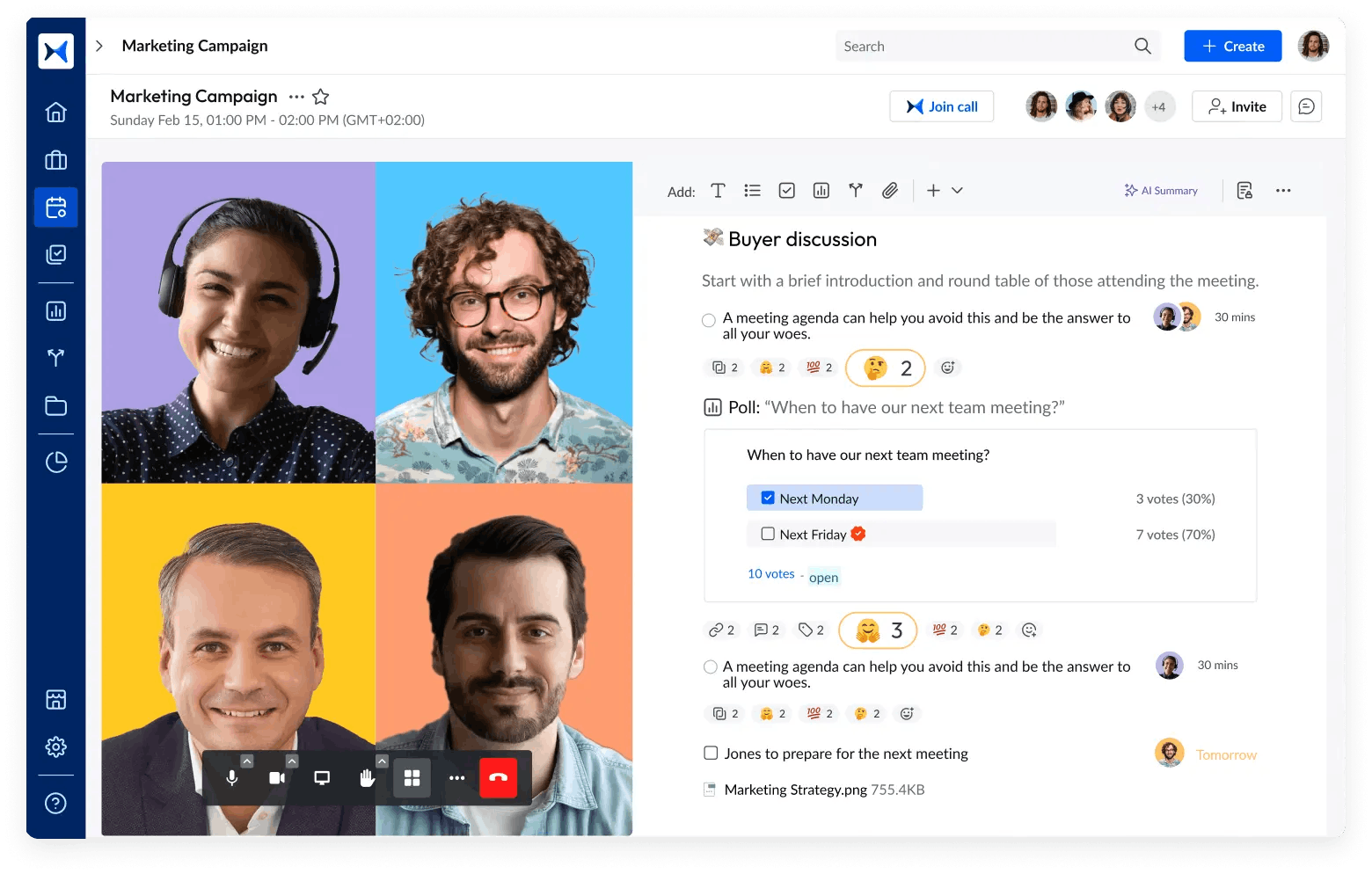
- Action tracking: ML-powered action tracking ensures all tasks discussed in previous meetings are monitored and linked to their respective outcomes. This helps teams proactively prepare follow-ups, avoiding delays and missed opportunities.
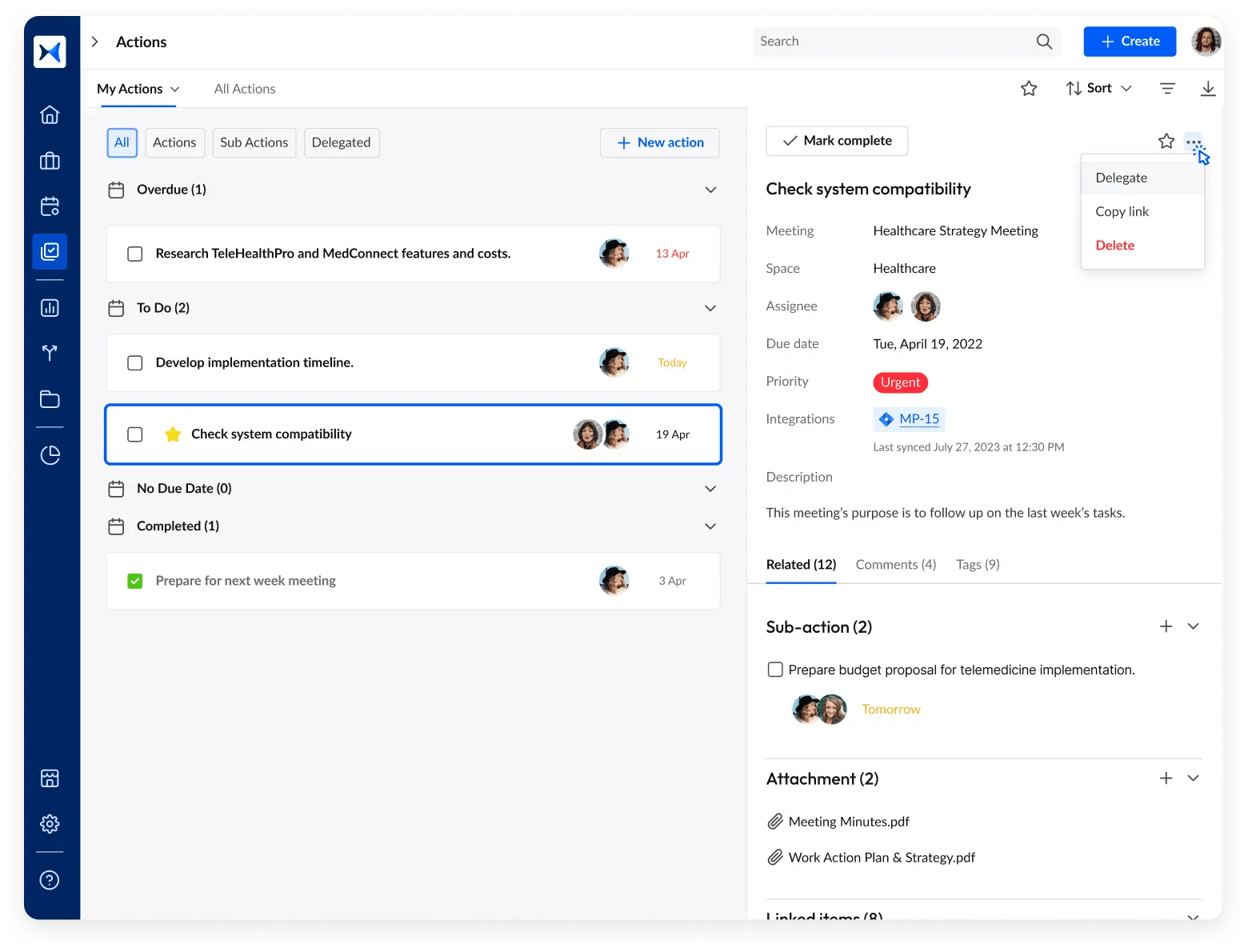
- Meeting minutes automation: During meetings, adam.ai’s machine learning algorithms generate real-time summaries and action items. This automation ensures accurate documentation and simplifies preparation for subsequent meetings.
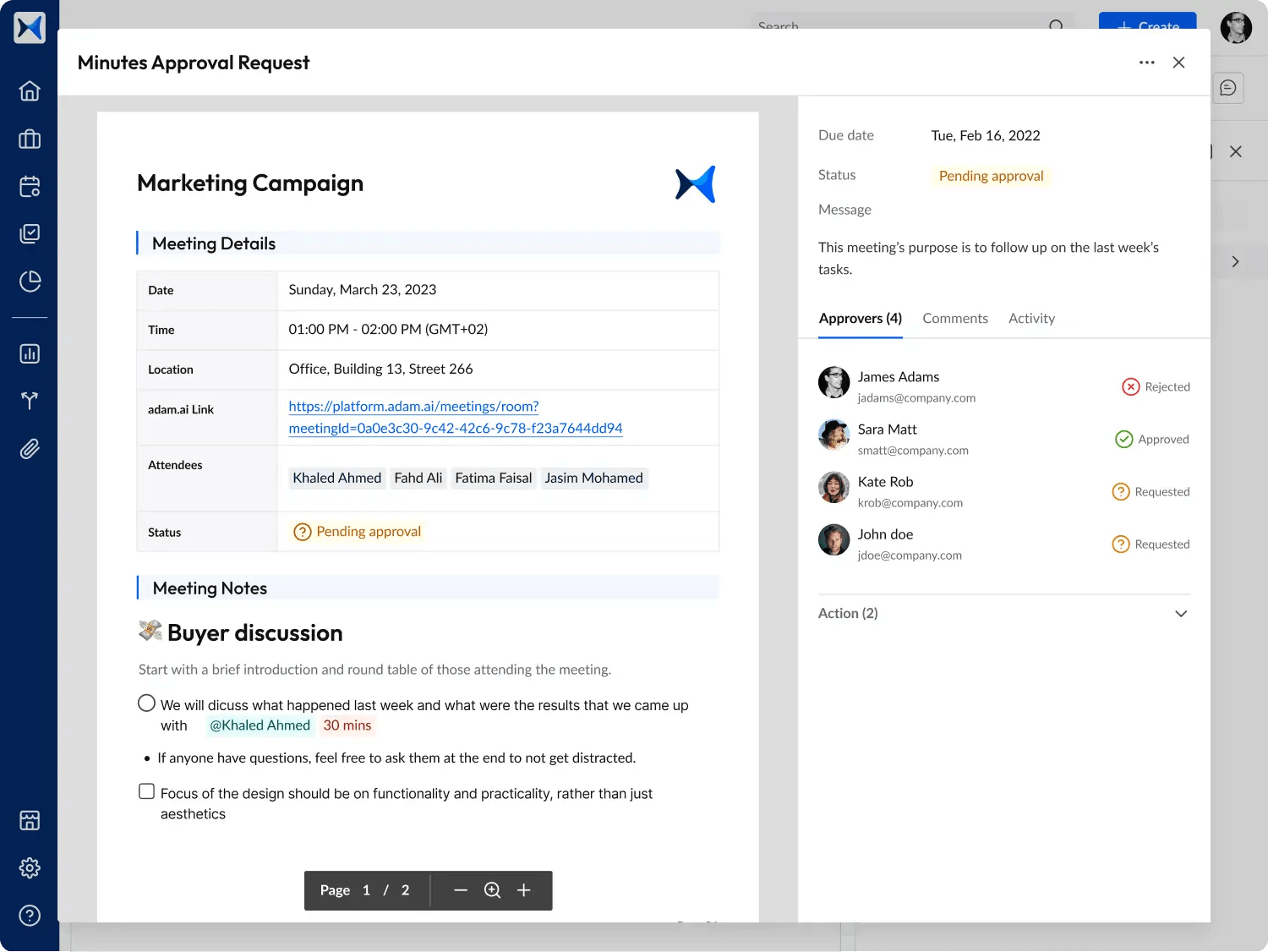
- Multi-space management: By categorizing and analyzing data from different spaces or projects, adam.ai personalizes preparation workflows for each team, ensuring agendas and insights are relevant to their specific needs.
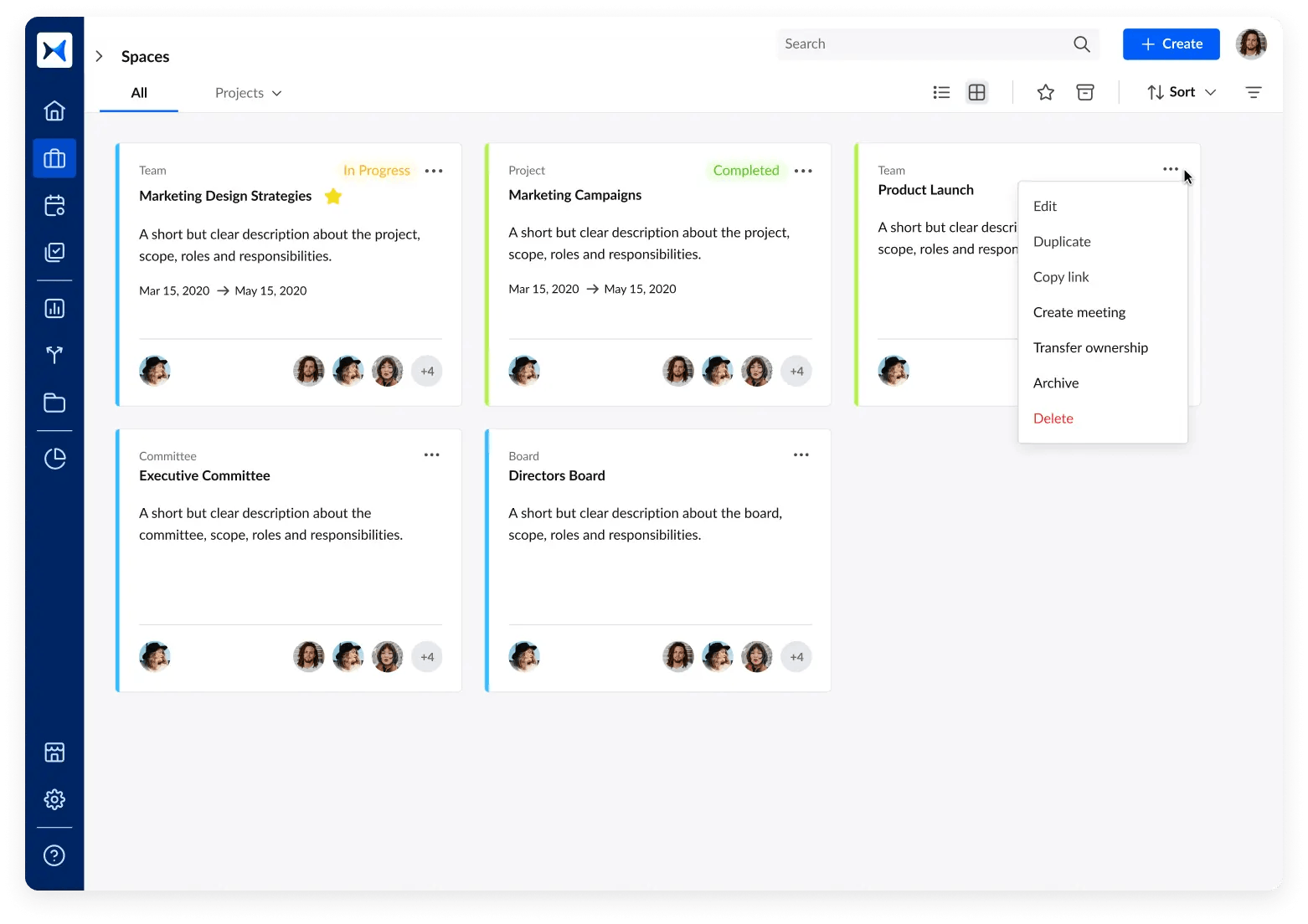
- Analytical dashboards: ML analyzes historical and current meeting data to provide insights on patterns, participation, and outcomes. These dashboards help teams identify areas for improvement and prepare more effectively for future meetings.
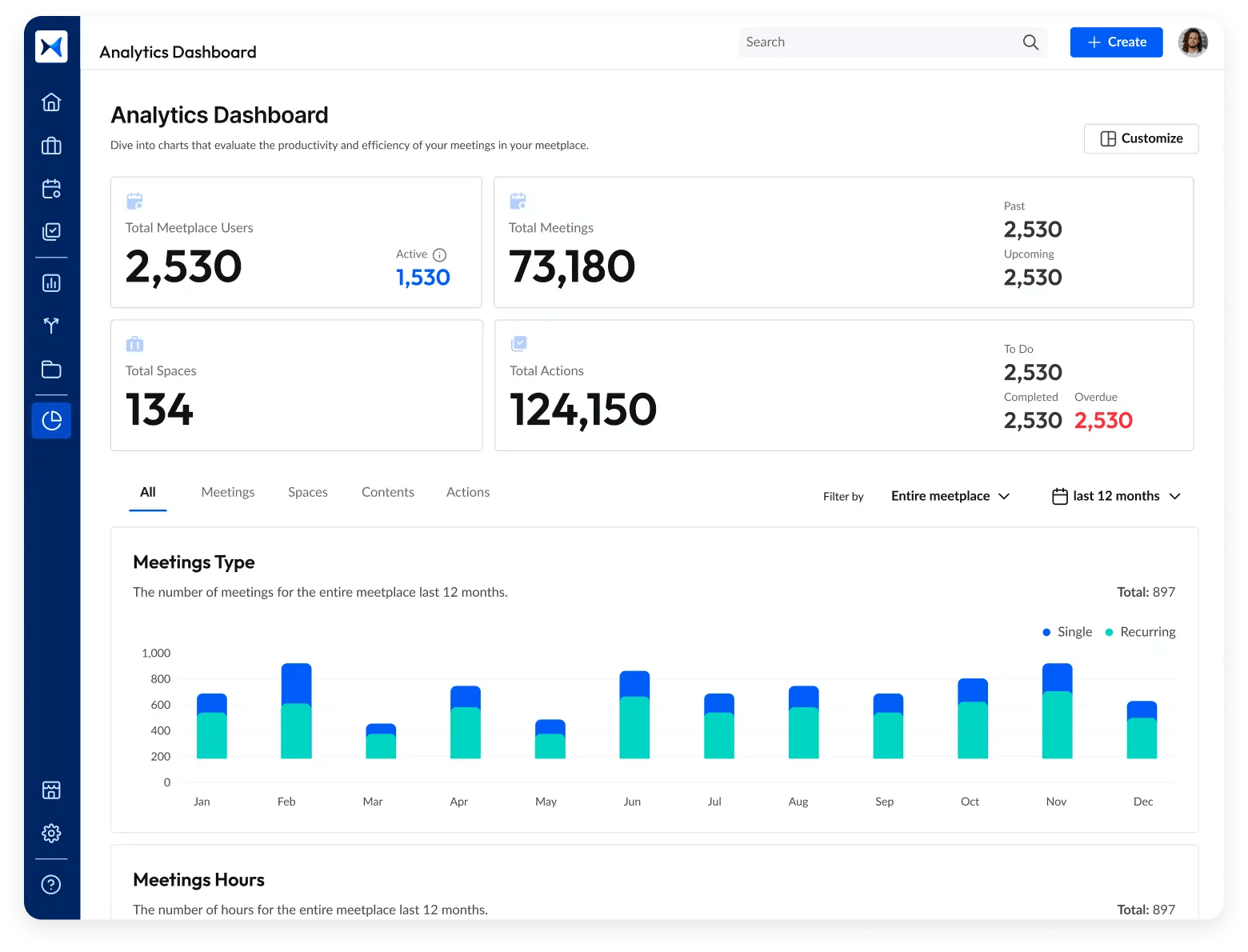
Transform how you conduct critical meetings—From meticulous preparation to effective execution and insightful follow-up, adam.ai integrates comprehensive analytics, full customization, and intuitive interfaces with powerful meeting management tools.
Easy onboarding. Enterprise-grade security. 24/7 dedicated support.
The bottom line
Integrating machine learning into meeting preparation unlocks new levels of efficiency and productivity. Tools like adam.ai leverage machine learning to tailor agendas, track actions, and centralize meeting resources, enabling organizations to prepare smarter and achieve greater outcomes.
And while there may be multiple solutions available, here is why adam.ai is the meeting management software platform you can trust:
- adam.ai is one of Atlassian Ventures' portfolio companies.
- In the meeting management software category on G2, adam.ai has been ranked a leader and a high performer for successive quarters in the past years.
- adam.ai has been included in the Forrester Report in the AI-enabled meeting technology landscape.
- adam.ai is trusted and used by powerful teams and organizations worldwide for all types of critical meetings, like board, committee, project management, and business development meetings.
- And most importantly, adam.ai integrates with your existing workflow, is SOC2 compliant, provides dedicated support and success, and has a free trial option.
Subscribe to adam.ai blog
Stay ahead with the latest insights—get our newest blog posts, tips, and updates sent straight to your inbox.