January 12, 2025 · 13 min read
Data-Driven Decision Making in Medical Project Meetings
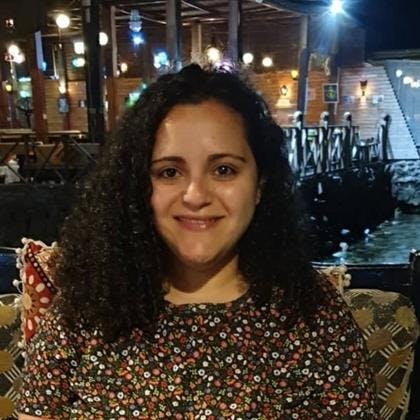
Shaimaa Badawi
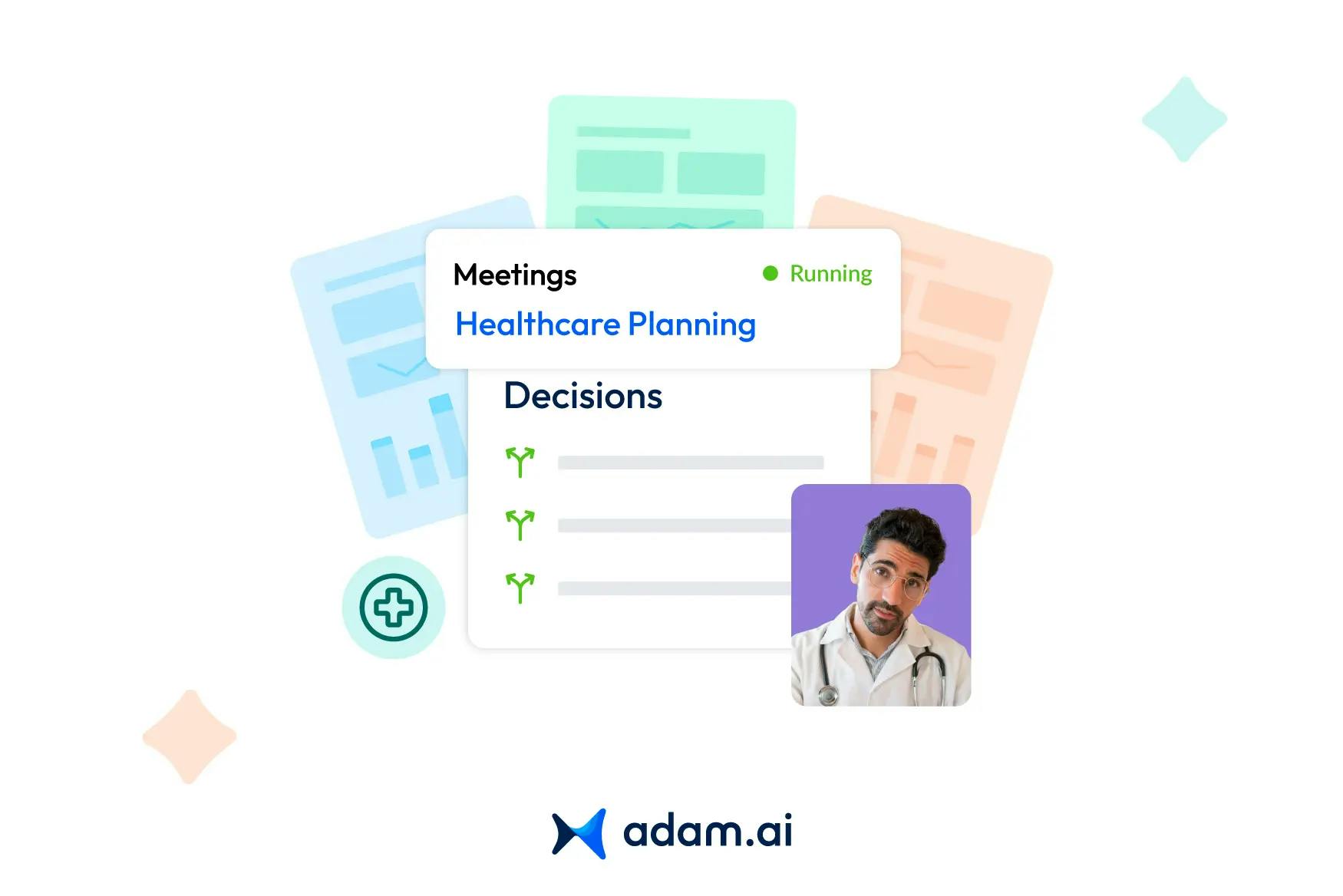
Data-driven decision-making is redefining how healthcare projects are planned, executed, and evaluated. With rising complexities in patient care and resource management, project meetings are becoming critical touchpoints for aligning teams and achieving impactful outcomes. In this article, we explore how incorporating data-driven strategies into medical project meetings can streamline collaboration, enhance decision-making, and ultimately lead to better results.
What is data-driven decision-making in medical project management?
Data-driven decision-making in medical project management is the process of using data as the foundation for planning, execution, and evaluation in healthcare projects. Instead of relying on intuition or traditional practices, this approach leverages actionable insights derived from data to guide decisions, ensuring they are objective, evidence-based, and aligned with project objectives.
Medical project management often involves complex variables such as patient care quality, resource allocation, and compliance with stringent regulations. Data-driven decision-making simplifies these challenges by integrating data from various sources, electronic health records, IoT devices, clinical trial results, and operational metrics, to create a comprehensive understanding of the project landscape.
For example, project managers can use predictive analytics to identify potential risks or delays and adjust timelines or resources accordingly.
Benefits of this approach
- Enhanced accuracy: By analyzing real-time and historical data, project managers can make precise decisions that reduce inefficiencies and errors.
- Improved outcomes: Tailoring strategies to the insights gained from data often leads to better patient outcomes and optimized project performance.
- Proactive planning: Data allows managers to anticipate challenges, allocate resources effectively, and mitigate risks before they escalate.
How does big data enhance decision-making in healthcare project meetings?
Big data plays a major role in healthcare project meetings by enabling teams to analyze vast, diverse, and real-time datasets to make informed, evidence-backed decisions. This integration of big data allows healthcare organizations to move beyond traditional decision-making methods. In multidisciplinary care meetings, data-driven approaches bring diverse expertise together to develop comprehensive healthcare solutions.
How big data enhances decision-making in healthcare project meetings
- Identifying trends and patterns: Big data consolidates information from electronic health records (EHRs), patient feedback, and operational logs, revealing patterns that inform resource allocation, staff management, and treatment protocols.
- Supporting predictive analytics: By analyzing historical data, project teams can forecast potential bottlenecks, risks, or patient demands. Machine learning enhances meeting preparation by analyzing past decisions and forecasting project needs. For instance, predictive models might identify a spike in demand for certain medical services, enabling proactive planning.
- Enhancing collaborative decision-making: With centralized dashboards and visualized data, project stakeholders can align their decisions, ensuring transparency and cohesion in meetings. AI-driven solutions also play a role in reducing meeting fatigue, making healthcare project discussions more productive.
- Improving patient outcomes: Insights derived from big data help prioritize patient-centric initiatives, from reducing hospital readmission rates to personalizing treatment plans based on historical data.
- Ensuring cost-effectiveness: Big data enables teams to track expenses in real time, helping optimize project budgets while reducing waste and inefficiencies.
What types of data are crucial for successful medical project management?
In medical project management, the success of a project often hinges on the quality and variety of data used for decision-making. Managing healthcare projects requires integrating multiple data types to ensure accurate planning, effective execution, and measurable outcomes. The diversity of data also allows for a holistic view of patient care, operational efficiency, and project alignment with strategic objectives.
Key data types crucial for success
1. Patient data
- Electronic health records (EHRs): Include patient demographics, medical histories, and treatment plans.
- Real-time monitoring data: Captured via IoT devices like wearable sensors and smart medical equipment. Telehealth and telemedicine initiatives can benefit from data-driven insights, improving access and care delivery.
2. Clinical data
- Results from clinical trials, diagnostic imaging, and laboratory reports.
- Genomic data for personalized medicine projects.
3. Operational data
- Staffing levels, scheduling, and resource utilization rates.
- Supply chain data for medical equipment and pharmaceutical management.
4. Financial data
- Cost breakdowns for treatments, equipment, and staffing.
- Budget tracking to monitor spending against allocated resources.
5. Regulatory and compliance data
- Information related to healthcare standards, legal requirements, and compliance protocols.
- Data from quality audits or inspections.
How can data analytics improve patient outcomes in medical projects?
Data analytics is crucial for improving patient outcomes in medical projects by turning raw data into actionable insights. It empowers healthcare teams to personalize care, predict complications, and optimize resources, leading to enhanced treatment efficacy and patient satisfaction.
How data analytics improves patient outcomes
- Personalized treatment plans: Advanced analytics tools process patient-specific data, such as medical history, genetic information, and current health conditions, to create tailored treatment plans. This ensures patients receive the most effective interventions.
- Predictive healthcare: Analytics can predict potential complications or disease progressions by analyzing historical and real-time data. For instance, predictive models can identify high-risk patients for early intervention, reducing hospitalizations.
- Improved care coordination: Data analytics helps integrate information from different departments, creating a unified view of a patient's journey. This enhances communication among care teams, minimizing errors and redundant procedures. Meeting management platforms can streamline patient care coordination by ensuring data accessibility and clear communication across departments.
- Faster clinical decisions: Real-time analytics allows medical teams to access up-to-date information during critical moments, enabling quicker and more informed decisions in patient care.
- Enhanced monitoring and follow-up: Analytics tools paired with IoT devices facilitate continuous monitoring of patients’ health. Alerts for irregularities, such as abnormal heart rates, help doctors intervene promptly.
What are the challenges of implementing data-driven strategies in healthcare projects?
Implementing data-driven strategies in healthcare projects can lead to transformative improvements, but the process comes with significant challenges. These obstacles often arise from the complexity of healthcare systems, the sensitivity of patient data, and the need for cultural and technological adaptation.
Key challenges
1. Data privacy and security concerns
- Protecting sensitive patient information is paramount, yet ensuring compliance with regulations like HIPAA or GDPR can be complex and resource-intensive.
- Data breaches or unauthorized access can undermine trust and lead to legal consequences.
2. Fragmented data systems
- Many healthcare organizations struggle with data silos, where critical information is stored across disconnected systems that don’t communicate effectively.
- Integrating disparate sources, such as EHRs, clinical trials, and IoT devices, is a logistical and technical challenge.
3. Data quality issues
- Incomplete, outdated, or inaccurate data can lead to flawed analysis and misguided decisions.
- Standardizing data formats and maintaining data integrity require continuous effort.
4. Resistance to change
- Shifting from traditional, intuition-based decision-making to data-driven approaches often meets resistance from healthcare professionals who are accustomed to established methods. Strong leadership and effective management are crucial for implementing data-driven strategies in healthcare projects.
- Training and culture shifts are necessary but can be time-consuming. Smart meeting agendas powered by data ensure that healthcare projects stay focused on critical objectives.
5. Cost and resource constraints
- Implementing advanced analytics systems and hiring skilled data professionals require substantial financial investment.
- Smaller healthcare facilities or projects may lack the resources to adopt such systems effectively.
6. Ethical considerations
- Balancing data use with ethical principles, such as informed consent and unbiased decision-making, adds complexity to data-driven strategies.
- Decisions based solely on algorithms may miss nuances in patient care.
7. Technical expertise gaps
- A shortage of skilled professionals capable of analyzing and interpreting complex datasets can slow adoption.
- Training existing staff or hiring specialized personnel is often a lengthy and expensive process.
How can healthcare enterprises overcome barriers to adopting data-driven methodologies?
Healthcare enterprises can overcome barriers to adopting data-driven methodologies by implementing strategic initiatives that address organizational, technical, and cultural challenges. A thoughtful approach ensures the smooth integration of data-driven processes and maximizes their potential for improved patient outcomes and operational efficiency.
Practical steps to overcome adoption barriers
1. Invest in infrastructure
- Upgrade legacy systems to advanced analytics platforms that support interoperability and scalability.
- Implement centralized data warehouses or cloud-based solutions to streamline data storage and access.
2. Foster a culture of data-driven decision-making
- Engage leadership to champion data initiatives and emphasize their value.
- Provide training programs to enhance data literacy among staff, enabling them to understand and use analytics tools effectively.
3. Enhance data integration and management
- Break down data silos by ensuring systems are interoperable and can share information seamlessly across departments.
- Standardize data formats and implement rigorous data quality protocols to ensure accuracy and reliability.
4. Prioritize data security and compliance
- Invest in advanced cybersecurity measures such as encryption, multi-factor authentication, and regular audits.
- Ensure adherence to regulations like HIPAA and GDPR to maintain patient trust and avoid legal issues.
5. Leverage partnerships and collaborations
- Collaborate with technology vendors, data scientists, and consultants to accelerate the adoption process. AI-powered meeting tools can streamline discussions, track tasks, and ensure project goals remain a priority.
- Join healthcare consortiums to share knowledge, best practices, and resources.
6. Start with pilot projects
- Test data-driven methodologies on smaller, manageable projects to demonstrate their benefits and build confidence among stakeholders.
- Use the insights gained from these pilots to scale up and refine processes.
7. Communicate value with measurable outcomes
- Track key performance indicators (KPIs) such as cost savings, operational efficiency, and patient outcomes to showcase the impact of data-driven strategies.
- Share success stories internally to build momentum and support for wider adoption.
What role does technology play in medical data collection and analytics for project success?
Technology plays a transformative role in medical data collection and analytics, serving as the backbone for project success in healthcare. From gathering comprehensive datasets to deriving actionable insights, technology enables healthcare organizations to make evidence-based decisions that improve outcomes and streamline operations.
Key roles of technology
1. Automating data collection
- Technologies like electronic health records (EHRs) and IoT devices ensure seamless collection of patient data, including vital signs, diagnostic results, and treatment progress, in real time.
- Wearables and remote monitoring tools allow continuous data flow, reducing manual errors and improving accuracy.
2. Enabling interoperability
- Advanced systems ensure that data from disparate sources, such as labs, hospitals, and clinics, can be integrated and accessed through centralized platforms.
- Interoperable technologies break down silos, providing a holistic view of patient care and project progress.
3. Providing advanced analytics
- Tools powered by AI and machine learning analyze large, complex datasets to uncover trends, predict outcomes, and identify risks. AI in meeting management simplifies complex healthcare projects by automating data analysis and decision tracking.
- Predictive analytics can forecast patient needs or potential project roadblocks, enabling proactive management.
4. Supporting real-time decision-making
- Dashboards and visualization tools deliver real-time updates, empowering healthcare project teams to make quick, informed decisions during critical moments.
- Technologies like natural language processing (NLP) extract actionable insights from unstructured data, such as physician notes or research articles.
5. Enhancing collaboration
- Digital collaboration platforms allow teams to share data, insights, and updates seamlessly, improving coordination and communication across departments and stakeholders.
- Video conferencing and virtual meeting tools enable efficient discussions and decision-making, especially for distributed teams.
6. Improving data governance
- Technology ensures compliance with data privacy and security regulations through encryption, access controls, and regular audits.
- Automated data validation and cleaning tools enhance data quality, ensuring accuracy and reliability.
How do data-driven decisions align healthcare projects with strategic goals?
Data-driven decisions ensure that healthcare projects are strategically aligned by providing a clear, evidence-based framework for prioritizing actions, optimizing resources, and measuring outcomes. This alignment enables organizations to achieve both short- and long-term objectives efficiently while maintaining a patient-centered focus. Here's how:
1. Setting measurable objectives
- Data analytics helps project managers identify key performance indicators (KPIs) that are directly linked to organizational goals, such as reducing patient wait times or improving treatment efficacy.
- Continuous monitoring of these KPIs ensures that projects remain on track.
2. Optimizing resource allocation
- By analyzing operational data, organizations can allocate resources, such as staff, equipment, and funding, more effectively to high-priority areas.
- This ensures that projects focus on delivering maximum value with minimal waste. Optimizing meeting time with data-driven strategies increases ROI and reduces inefficiencies in healthcare projects.
3. Aligning with patient-centric goals
- Insights from patient data enable healthcare projects to align with the broader goal of improving care quality and outcomes, such as reducing hospital readmissions or enhancing preventive care.
4. Enhancing strategic foresight
- Predictive analytics helps organizations anticipate future challenges, such as demographic shifts or changes in disease prevalence, allowing for proactive adjustments to strategic plans.
5. Driving accountability
- Transparent data reporting ensures that all stakeholders, management, clinicians, and external partners, are aligned on project goals and their contributions toward achieving them.
- Regular data reviews foster a culture of accountability and continuous improvement.
6. Measuring and demonstrating ROI
- Data-driven decision-making provides concrete evidence of a project’s impact, such as cost savings, improved operational efficiency, or better patient outcomes.
- This data helps justify investments and guide future strategic initiatives.
What are the ethical considerations in leveraging big data for medical projects?
Leveraging big data in medical projects comes with significant ethical considerations, primarily due to the sensitivity of healthcare information and the potential implications for patients, healthcare providers, and society. Addressing these concerns is essential to ensure trust, compliance, and equitable outcomes.
Key ethical considerations
1. Patient privacy and confidentiality
- Big data often involves sensitive patient information, such as medical histories and genetic data. Ensuring this data is kept confidential and protected from unauthorized access is paramount.
- Organizations must comply with regulations like HIPAA or GDPR, which mandate strict privacy and security standards.
2. Informed consent
- Patients must be informed about how their data will be collected, analyzed, and used in medical projects.
- Obtaining explicit consent, especially for secondary uses like research or AI training, is critical to maintaining ethical standards.
3. Data security
- Advanced encryption, regular audits, and secure storage systems are necessary to protect data from breaches or misuse.
- Security lapses can result in significant harm to patients and damage to the organization’s reputation.
4. Bias and fairness
- Algorithms and datasets must be free from biases that could lead to unequal treatment of certain populations, such as underrepresented groups.
- Regular audits and diverse data sources can mitigate these risks and ensure fair outcomes.
5. Transparency and accountability
- Healthcare organizations must be transparent about how data is used, who has access to it, and the rationale behind data-driven decisions.
- Clear documentation and governance processes ensure accountability in data usage.
6. Balancing individual rights and public health
- Ethical dilemmas can arise when individual privacy conflicts with public health goals, such as during disease surveillance or pandemic response.
- Striking a balance between these priorities requires careful policy-making and stakeholder engagement.
7. Ownership and data sharing
- Questions about who owns healthcare data, patients, providers, or organizations, must be clearly addressed.
- Ethical data sharing practices involve anonymization, aggregation, and adherence to legal and ethical standards.
8. Potential misuse
- Big data could be exploited for purposes unrelated to patient care, such as discriminatory insurance practices or profit-driven decision-making.
- Clear guidelines and oversight are needed to prevent unethical exploitation.
How can adam.ai transform healthcare project meetings?
adam.ai can revolutionize healthcare project meetings by providing an integrated platform that addresses the complexities of data-driven decision-making. Its features enable healthcare teams to plan, collaborate, and execute projects more effectively, ensuring alignment with strategic goals and improved patient outcomes. Here's how:
- Agenda management: Structure project meetings with focused, data-driven agendas. Assign topics, speakers, and durations to ensure discussions stay on track and contribute to actionable outcomes.
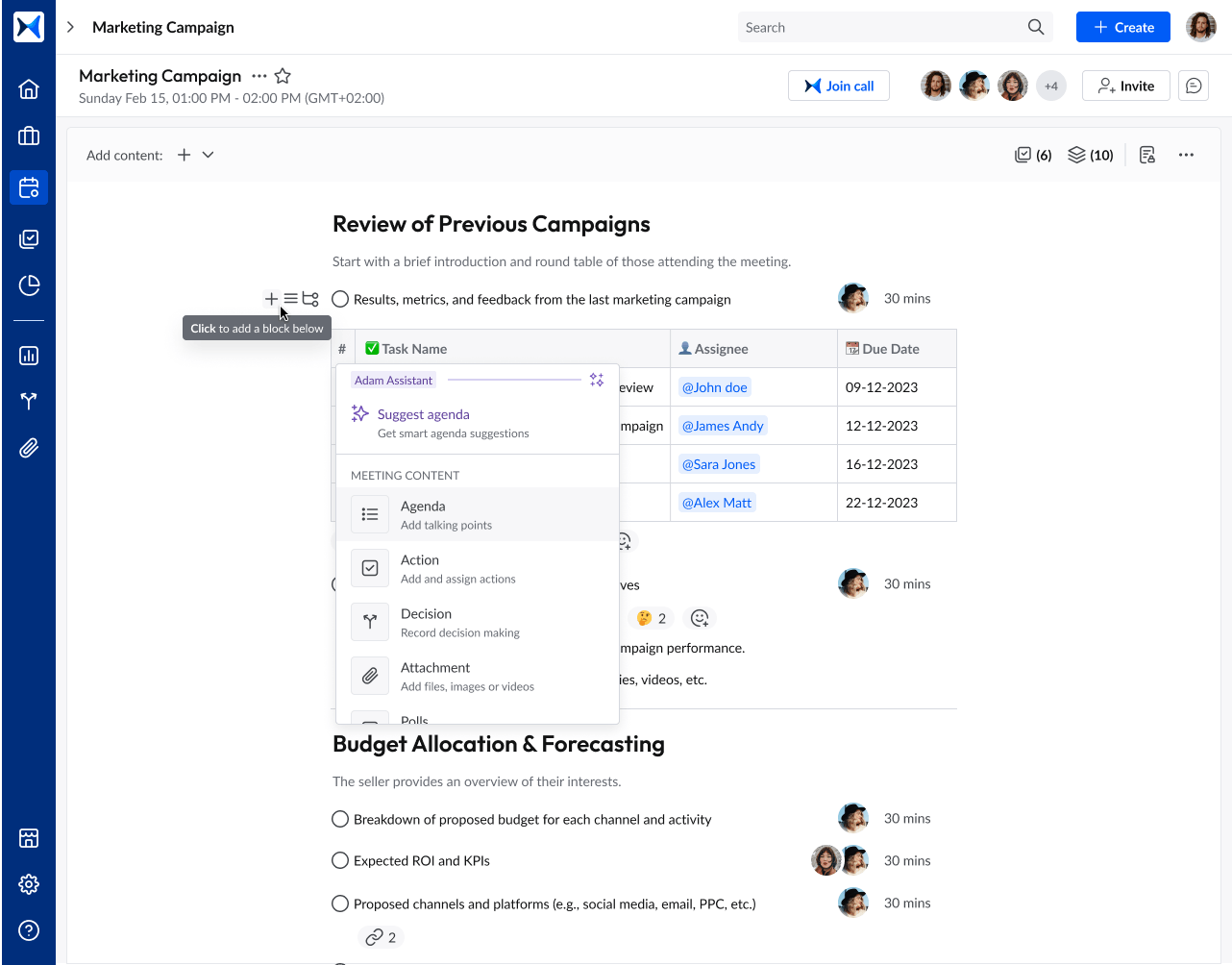
- Content collaboration: Centralize all relevant data, reports, and insights within the platform, making it easier for teams to access and share critical information during project meetings.
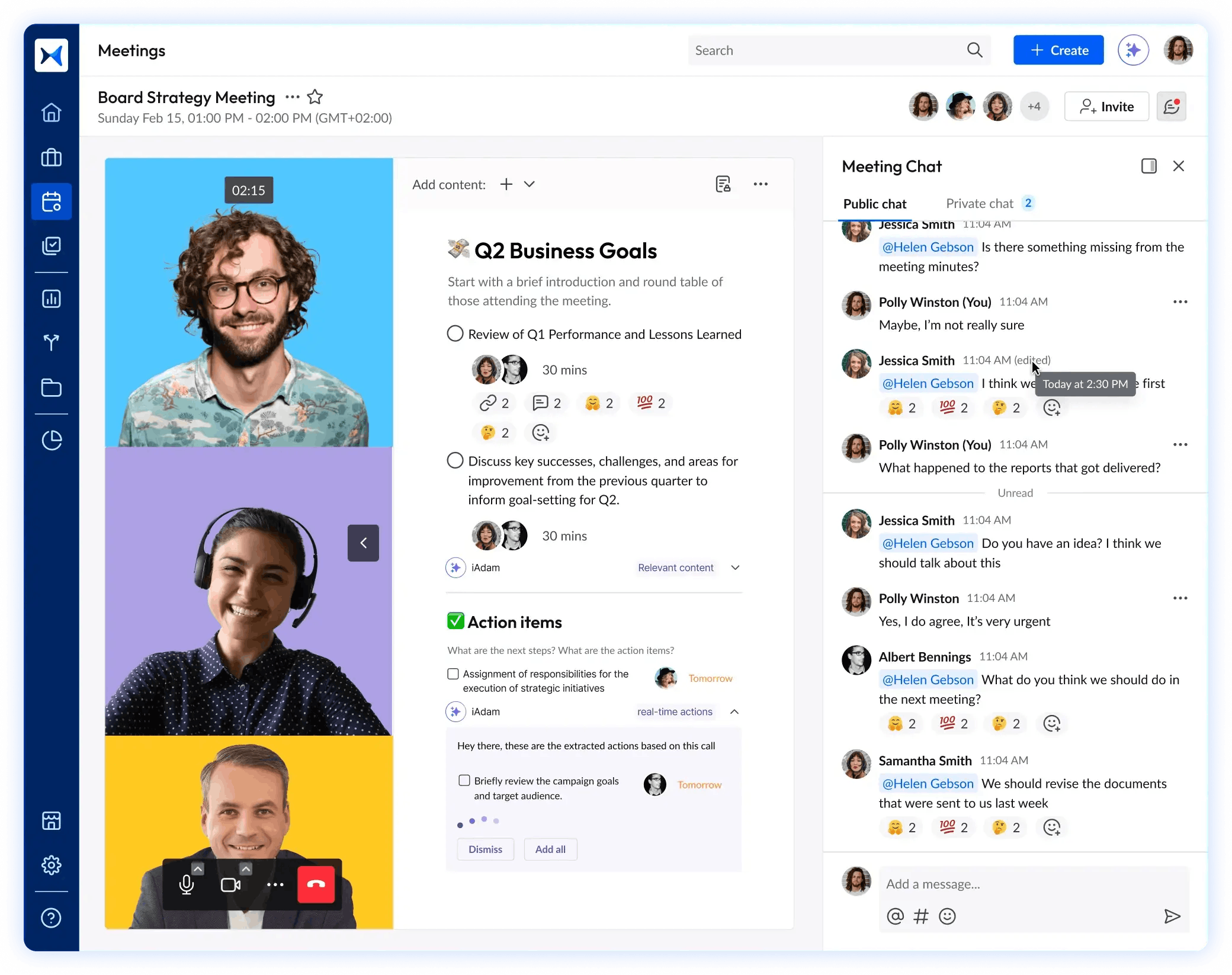
- Action tracking: Monitor and follow up on tasks assigned during meetings to ensure accountability and timely completion of healthcare initiatives.
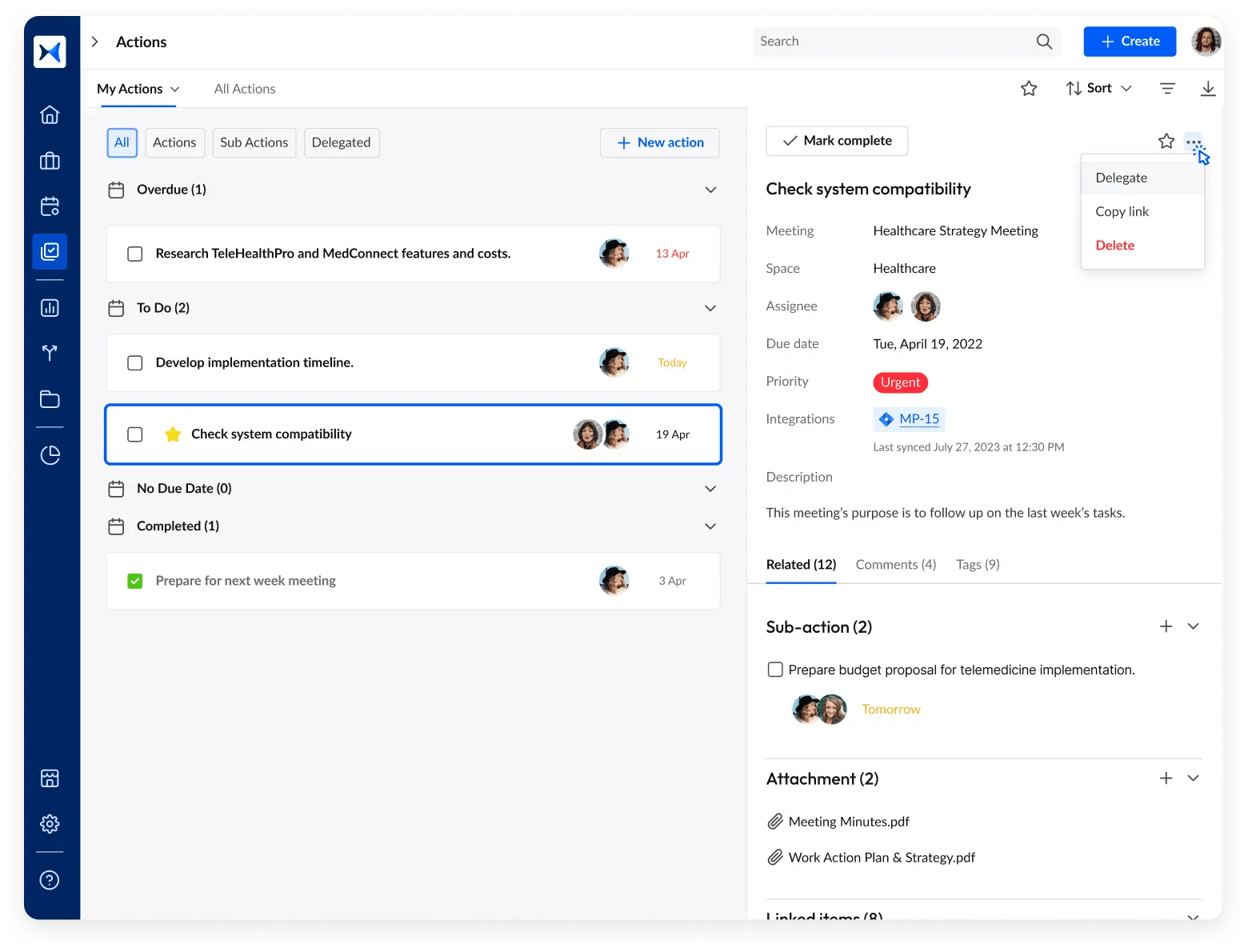
- Meeting minutes: Automatically generate, store, and share meeting minutes, capturing essential decisions and insights for reference and compliance purposes.
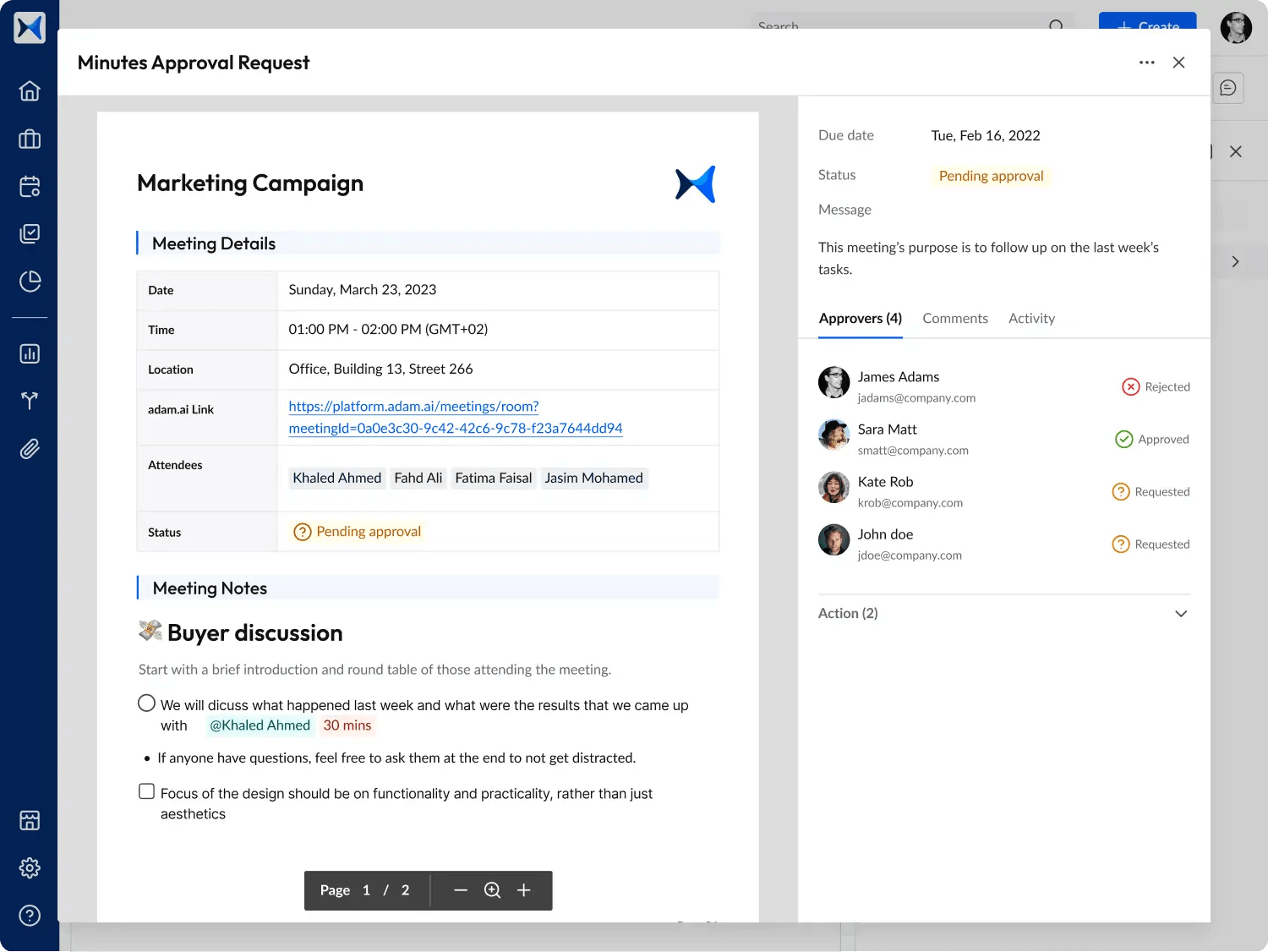
- Multi-space management: Organize discussions across various healthcare projects or departments, ensuring seamless collaboration among cross-functional teams.
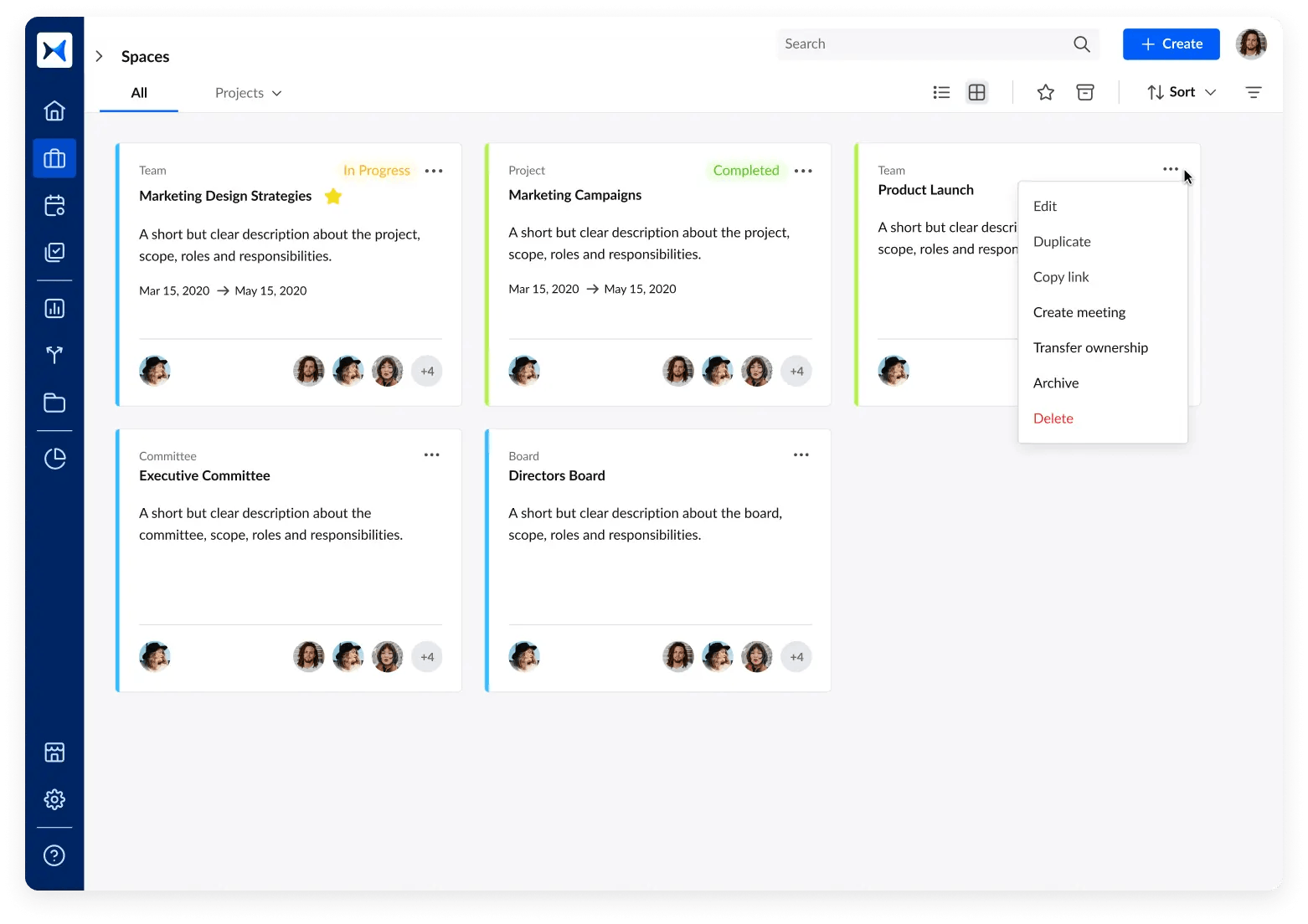
- Analytical dashboards: Gain real-time insights into project progress and meeting outcomes, empowering teams to make data-informed adjustments for improved results.
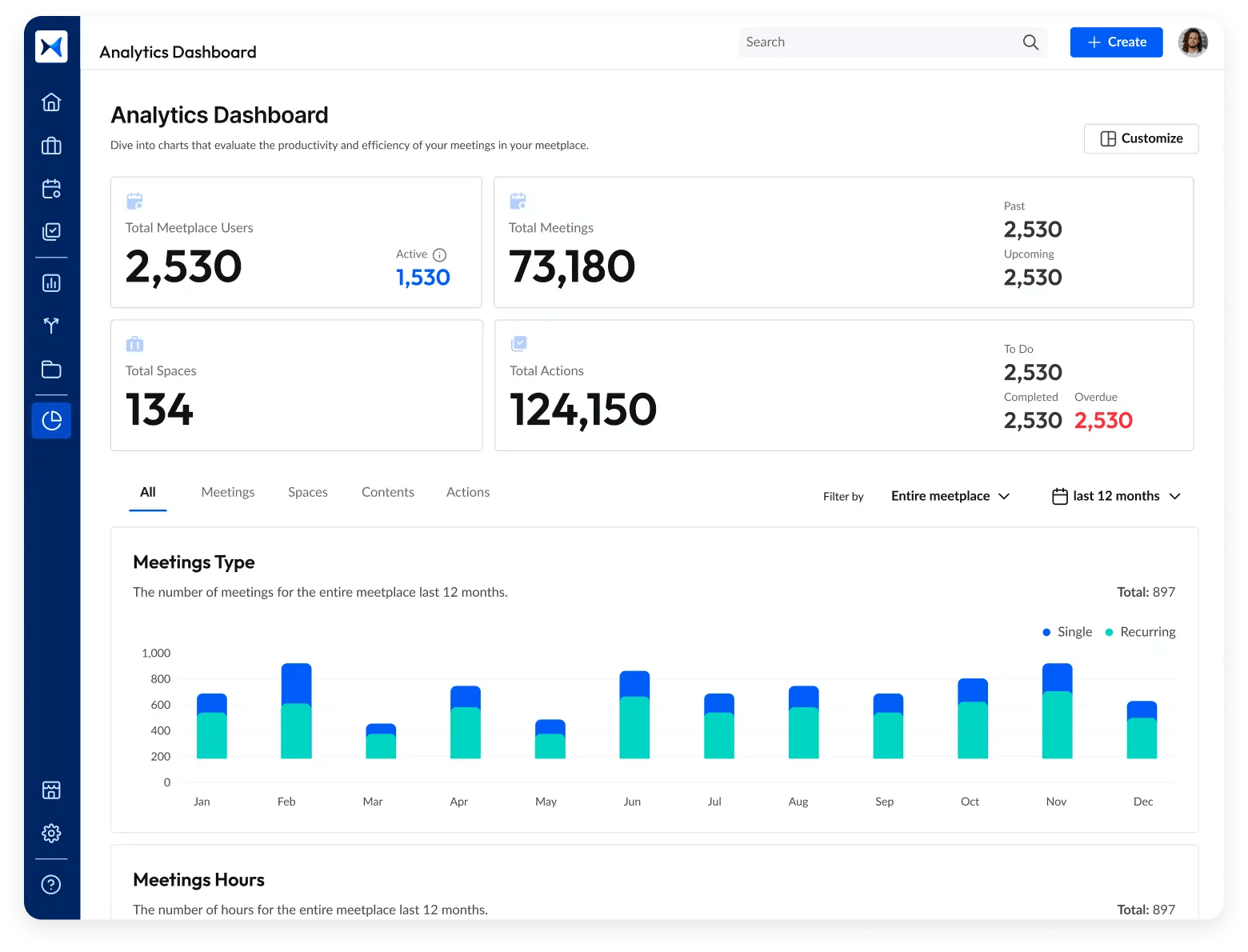
Transform how you conduct critical meetings—From meticulous preparation to effective execution and insightful follow-up, adam.ai integrates comprehensive analytics, full customization, and intuitive interfaces with powerful meeting management tools.
Easy onboarding. Enterprise-grade security. 24/7 dedicated support.
The bottom line
Data-driven decision-making empowers healthcare teams to navigate complex projects with clarity and precision. By integrating actionable insights and structured approaches into meetings using modern tools, organizations can achieve their goals while delivering exceptional patient care.
And while there may be multiple solutions available, here is why adam.ai is the meeting management software platform you can trust:
- adam.ai is one of Atlassian Ventures' portfolio companies.
- In the meeting management software category on G2, adam.ai has been ranked a leader and a high performer for successive quarters in the past years.
- adam.ai has been included in the Forrester Report in the AI-enabled meeting technology landscape.
- adam.ai is trusted and used by powerful teams and organizations worldwide for all types of critical meetings, like board, committee, project management, and business development meetings.
- And most importantly, adam.ai integrates with your existing workflow, is SOC2 compliant, provides dedicated support and success, and has a free trial option.
Subscribe to adam.ai blog
Stay ahead with the latest insights—get our newest blog posts, tips, and updates sent straight to your inbox.